Massive Imaginative and prescient-Language Fashions (LVLMs) have made vital strides lately, but a number of key limitations persist. One main problem is aligning these fashions successfully with human expectations, notably for duties involving detailed and exact visible data. Historically, LVLMs bear a two-stage coaching paradigm: pretraining adopted by supervised fine-tuning. Nevertheless, supervised fine-tuning alone can’t totally overcome limitations, such because the shortage and excessive price related to producing large-scale, human-annotated choice datasets. Furthermore, standard reinforcement studying strategies require costly reward fashions that will not totally seize the nuanced and subjective nature of human suggestions.
A workforce of researchers from China suggest Imaginative and prescient-R1: a novel vision-guided R1-like reinforcement studying algorithm for LVLMs that rewards fashions with definitive imaginative and prescient suggestions. Imaginative and prescient-R1 leverages curated instruction knowledge, thereby eliminating the dependency on specialised reward fashions and handcrafted choice datasets. Central to this technique is a criterion-driven reward perform, which offers complete evaluations of mannequin completions primarily based on particular visible job standards. Moreover, a progressive rule refinement technique is employed, dynamically adjusting reward standards all through the coaching course of. This strategy ensures steady efficiency enchancment, successfully mitigating reward hacking points and selling extra correct object localization.
The Imaginative and prescient-R1 algorithm incorporates a number of essential technical improvements. First, the criterion-driven reward perform consists of twin format rewards, recall rewards, and precision rewards. Twin format rewards guarantee outputs adhere strictly to template and content material constraints, important for dependable object detection duties. The recall reward emphasizes the mannequin’s capability to establish all related situations, essential for avoiding omissions in predictions. The precision reward encourages high-quality bounding field predictions by calculating the typical Intersection over Union (IoU) of legitimate predictions. Moreover, the progressive rule refinement technique is impressed by curriculum studying rules, steadily rising coaching issue by staged development and differentiation insurance policies, thereby fostering sturdy and generalized studying.
Experiments carried out utilizing two state-of-the-art LVLMs, Griffon-G-7B and Qwen2.5-VL-7B, display the sturdy capabilities of Imaginative and prescient-R1. Outcomes on in-domain datasets equivalent to MSCOCO and ODINW-13 present vital efficiency enhancements. Particularly, Imaginative and prescient-R1 improves Griffon-G-7B’s mAP scores by 2.5% on common throughout various duties. Extra impressively, Imaginative and prescient-R1 boosts Qwen2.5-VL-7B’s efficiency considerably, displaying an 8.9% enchancment in COCO object detection duties and reaching superior scores in comparison with its bigger, 72B counterpart. On difficult out-of-domain localization duties, Imaginative and prescient-R1 persistently outperforms supervised fine-tuning (SFT), demonstrating its sturdy generalization capabilities and robustness in advanced situations.
In conclusion, Imaginative and prescient-R1 introduces an revolutionary reinforcement studying strategy tailor-made for LVLMs that successfully addresses current alignment points with out requiring expensive annotated datasets or advanced reward modeling. Its criterion-driven reward construction and progressive rule refinement technique not solely improve the accuracy and comprehensiveness of object localization duties but additionally considerably enhance generalization to unseen situations. The profitable integration of Imaginative and prescient-R1 with modern LVLM architectures highlights its potential to function a foundational technique, considerably advancing the state-of-the-art in vision-language understanding and sensible deployment in real-world functions.
Check out the Paper and GitHub Page. All credit score for this analysis goes to the researchers of this undertaking. Additionally, be at liberty to observe us on Twitter and don’t overlook to hitch our 85k+ ML SubReddit.
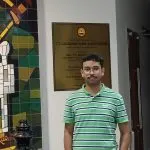
Sana Hassan, a consulting intern at Marktechpost and dual-degree pupil at IIT Madras, is enthusiastic about making use of know-how and AI to handle real-world challenges. With a eager curiosity in fixing sensible issues, he brings a recent perspective to the intersection of AI and real-life options.