Massive language fashions have remodeled how machines comprehend and generate textual content, particularly in complicated problem-solving areas like mathematical reasoning. These methods, referred to as R1-like fashions, are designed to emulate sluggish and deliberate thought processes. Their key energy is dealing with intricate duties requiring step-by-step reasoning throughout lengthy sequences. These capabilities make them beneficial for purposes comparable to fixing Olympiad-level math issues or logical reasoning duties, the place depth and coherence of reasoning are important.
A major problem in coaching these fashions is the in depth computation for reinforcement studying utilizing lengthy context home windows. Duties that require multi-step logic pressure fashions to supply lengthy outputs which consumes extra sources and slows down studying. Additional, not all lengthy responses contribute meaningfully to accuracy; many embrace redundant reasoning. These inefficiencies in response technology and excessive GPU utilization make it tough to successfully scale coaching, notably when working with fashions with 1.5 billion parameters.
Earlier makes an attempt to deal with this challenge embrace fashions like DeepScaleR, which makes use of a staged context size extension technique throughout coaching. DeepScaleR begins with an 8K context window and expands progressively to 24K over three coaching phases. Though this method helps information the mannequin to handle longer reasoning chains effectively, it nonetheless calls for roughly 70,000 A100 GPU hours. DeepScaleR reduces that to three,800 hours by means of a progressive technique however nonetheless requires appreciable {hardware}, together with setups with as much as 32 GPUs in some levels. This reveals that whereas enhancements are doable, the answer stays expensive and complicated.
Researchers at Tencent launched a technique known as FASTCURL to beat the inefficiencies of conventional reinforcement studying coaching. This methodology presents a curriculum-based technique aligned with context window enlargement. FASTCURL splits the dataset based mostly on enter immediate size into quick, lengthy, and mixed classes. The coaching progresses in 4 levels, every utilizing a unique dataset and context window setting. This method ensures the mannequin learns easy reasoning earlier than advancing to longer, extra complicated reasoning steps. The researchers emphasize that your complete coaching course of runs on a single node with simply 8 GPUs, decreasing setup complexity.
The method entails a deliberate segmentation of information by enter size, pushed by the speculation that longer prompts often result in longer and extra complicated outputs. The mannequin first learns utilizing quick prompts below an 8K window. As coaching proceeds, the mannequin transitions to a combined dataset with 16K window size, then to the lengthy dataset with the identical window measurement, and eventually opinions the mixed knowledge once more. Every stage is skilled for one iteration, and FASTCURL requires about 860 coaching steps. That is environment friendly in comparison with DeepScaleR’s 1,750 steps, representing a 50% discount in coaching time and useful resource utilization whereas sustaining effectiveness.
In efficiency evaluations, FASTCURL-1.5B-Preview confirmed enhancements over different fashions throughout 5 benchmarks. It scored 88.0 on MATH 500, 43.1 on AIME 2024, 74.2 on AMC 2023, 31.6 on Minerva Math, and 50.4 on OlympiadBench, with a mean PASS@1 rating of 57.5. In comparison with DeepScaleR-1.5B-Preview, which scored a mean of 57.0, FASTCURL carried out higher in 4 of 5 datasets. These outcomes spotlight that FASTCURL can outperform present methods whereas consuming considerably fewer sources. The mannequin additionally confirmed higher generalization, notably on datasets like AMC 2023 and Minerva Math, indicating robustness.
The analysis clearly outlines a computational drawback in coaching R1-like reasoning fashions and provides an progressive curriculum technique as an answer. The tactic supplies an environment friendly and sensible coaching framework by combining input-based knowledge segmentation with context enlargement. FASTCURL delivers sturdy efficiency utilizing fewer steps and restricted {hardware}, proving that strategic coaching design could be as highly effective as uncooked computational scale.
Check out the Paper. All credit score for this analysis goes to the researchers of this undertaking. Additionally, be at liberty to comply with us on Twitter and don’t neglect to affix our 85k+ ML SubReddit.
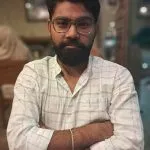
Nikhil is an intern advisor at Marktechpost. He’s pursuing an built-in twin diploma in Supplies on the Indian Institute of Know-how, Kharagpur. Nikhil is an AI/ML fanatic who’s at all times researching purposes in fields like biomaterials and biomedical science. With a powerful background in Materials Science, he’s exploring new developments and creating alternatives to contribute.