Integrating imaginative and prescient and language processing in AI has change into a cornerstone for growing programs able to concurrently understanding visible and textual information, i.e., multimodal information. This interdisciplinary subject focuses on enabling machines to interpret photographs, extract related textual info, and discern spatial and contextual relationships. These capabilities promise to reshape real-world purposes by bridging the visible and linguistic understanding hole from autonomous autos to superior human-computer interplay programs.
Regardless of many accomplishments within the subject, it has notable challenges. Many fashions prioritize high-level semantic understanding of photographs, capturing total scene descriptions however usually overlooking detailed pixel or region-level info. This omission undermines their efficiency in specialised duties requiring intricate comprehension, comparable to textual extraction from photographs or understanding spatial object relationships. Additionally, integrating a number of imaginative and prescient encoders to handle these points usually leads to computational inefficiency, growing coaching and deployment complexity.
Instruments like CLIP have traditionally set a benchmark for aligning visible and textual representations utilizing contrastive pretraining. Whereas efficient for basic duties, CLIP’s reliance on single-layer semantic options limits its adaptability to various challenges. Superior approaches have launched self-supervised and segmentation fashions that deal with particular duties, but they continuously depend on a number of encoders, which might enhance the computational calls for. These limitations spotlight the necessity for a flexible and environment friendly strategy that balances generalization and task-specific precision.
Researchers from the College of Maryland and Microsoft launched Florence-VL, a novel structure to handle these challenges and improve vision-language integration. This mannequin employs a generative imaginative and prescient basis encoder, Florence-2, to supply task-specific visible representations. This encoder departs from conventional strategies by using a prompt-based strategy, enabling it to tailor its options to varied duties comparable to picture captioning, object detection, and optical character recognition (OCR).
Central to Florence-VL’s effectiveness is its Depth-Breadth Fusion (DBFusion) mechanism, which integrates visible options throughout a number of layers and prompts. This twin strategy ensures the mannequin captures granular and high-level particulars, catering to various vision-language duties. Depth options are derived from hierarchical layers, providing detailed visible insights, whereas breadth options are extracted utilizing task-specific prompts, making certain adaptability to varied challenges. Florence-VL combines these options effectively by using a channel-based fusion technique, sustaining computational simplicity with out sacrificing efficiency. In depth coaching on 16.9 million picture captions and 10 million instruction datasets additional optimizes the mannequin’s capabilities. Not like conventional fashions that freeze sure elements throughout coaching, Florence-VL fine-tunes its complete structure throughout pretraining, attaining enhanced alignment between visible and textual modalities. Its instruction-tuning part refines its skill to adapt to downstream duties, supported by high-quality datasets curated for particular purposes.
Florence-VL has been examined throughout 25 benchmarks, together with visible query answering, OCR, and chart comprehension duties. It achieved an alignment lack of 2.98, considerably surpassing fashions comparable to LLaVA-1.5 and Cambrain-8B. The Florence-VL 3B variant excelled in 12 out of 24 evaluated duties, whereas the bigger 8B model constantly outperformed rivals. Its outcomes on OCRBench and InfoVQA benchmarks underline its skill to extract and interpret textual info from photographs with unparalleled precision.
Key takeaways from the analysis on Florence-VL are as follows:
- Unified Imaginative and prescient Encoding: A single imaginative and prescient encoder reduces complexity whereas sustaining task-specific adaptability.
- Job-Particular Flexibility: The prompt-based mechanism helps various purposes, together with OCR and grounding.
- Enhanced Fusion Technique: DBFusion ensures a wealthy mixture of depth and breadth options, capturing granular and contextual particulars.
- Superior Benchmark Outcomes: Florence-VL leads efficiency in 25 benchmarks, attaining an alignment lack of 2.98.
- Coaching Effectivity: Tremendous-tuning the complete structure throughout pretraining enhances multimodal alignment, yielding higher process outcomes.

In conclusion, Florence-VL addresses the vital limitations of present vision-language fashions by introducing an modern strategy that successfully combines granular and high-level visible options. The multimodal mannequin ensures task-specific adaptability by leveraging Florence-2 as its generative imaginative and prescient encoder and using the Depth-Breadth Fusion (DBFusion) mechanism whereas sustaining computational effectivity. Florence-VL excels throughout various purposes, comparable to OCR and visible query answering, attaining superior efficiency throughout 25 benchmarks.
Try the Paper, Demo, and GitHub Page. All credit score for this analysis goes to the researchers of this venture. Additionally, don’t neglect to observe us on Twitter and be part of our Telegram Channel and LinkedIn Group. When you like our work, you’ll love our newsletter.. Don’t Neglect to hitch our 60k+ ML SubReddit.
🚨 [Must Attend Webinar]: ‘Transform proofs-of-concept into production-ready AI applications and agents’ (Promoted)
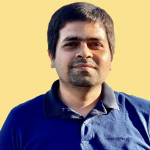
Asif Razzaq is the CEO of Marktechpost Media Inc.. As a visionary entrepreneur and engineer, Asif is dedicated to harnessing the potential of Synthetic Intelligence for social good. His most up-to-date endeavor is the launch of an Synthetic Intelligence Media Platform, Marktechpost, which stands out for its in-depth protection of machine studying and deep studying information that’s each technically sound and simply comprehensible by a large viewers. The platform boasts of over 2 million month-to-month views, illustrating its recognition amongst audiences.