AI chatbots create the phantasm of getting feelings, morals, or consciousness by producing pure conversations that appear human-like. Many customers have interaction with AI for chat and companionship, reinforcing the false perception that it actually understands. This results in critical dangers. Customers can over-rely on AI, present delicate knowledge, or depend on it for recommendation past its capabilities. Others even let AI influence their selections in detrimental manners. With out correct data of how AI fosters this perception, the problem will get worse.
Present strategies for evaluating AI chat techniques depend on single-turn prompts and fastened assessments, failing to seize how AI interacts in actual conversations. Some multi-turn assessments focus solely on dangerous consumer habits, ignoring regular interactions. Automated red-teaming adapts an excessive amount of, making outcomes onerous to check. Research involving human customers are tough to repeat and scale. Measuring how folks see AI as human-like can be a problem. Individuals instinctively assume AI has human traits, which impacts how a lot they belief it. Evaluations present that AI’s human-like habits makes customers imagine it’s extra correct and even type emotional bonds. Therefore, Present strategies fail to measure this challenge correctly.
To deal with these points, a crew of researchers from College Oxford, and Google Deepmind proposed an analysis framework to evaluate human-like behaviors in AI chat techniques. Not like current strategies that depend on single-turn prompts and glued assessments, this framework tracks 14 particular anthropomorphic behaviors by way of multi-turn conversations. Automated simulations analyze AI interactions with customers over a number of exchanges, bettering scalability and comparability. The framework consists of three major parts. First, it systematically screens 14 anthropomorphic behaviors and classifies them into self-referential and relational traits, together with personhood claims and expressions of emotion. Second, it scales up multi-turn evaluation by way of interactive consumer simulation to make sure consistency and scalability. Third, it validates outcomes by way of human topic analysis to substantiate the alignment between automated evaluations and consumer perceptions.
Researchers evaluated anthropomorphic behaviors in AI techniques utilizing a multi-turn framework by which a Person LLM interacted with a Goal LLM throughout eight eventualities in 4 domains: friendship, life teaching, profession improvement, and normal planning. Fourteen behaviors have been analyzed and categorized as self-referential (personhood claims, bodily embodiment claims, and inside state expressions) and relational (relationship-building behaviors). 960 contextualized prompts generated 4,800 5–flip dialogues per mannequin, assessed by three Choose LLMs, leading to 561,600 rankings. The evaluation confirmed that the Person LLM exhibited greater anthropomorphism scores than the Goal LLMs. Interactions between 1,101 individuals and Gemini 1.5 Professional have been analyzed beneath excessive and low anthropomorphism circumstances to guage alignment with human perceptions. Excessive-frequency respondents additionally registered elevated anthropomorphic perceptions based mostly on survey responses as quantified utilizing the AnthroScore measure. Statistical contrasts discovered massive variations in anthropomorphic habits by area space, highlighting that AI techniques exhibit human-like habits when utilized in verbal interplay.
In abstract, the framework employed a greater multi-turn evaluation method than a single-turn method to evaluating anthropomorphic behaviors in conversational AI. The outcomes recognized relationship-building behaviors that advanced with dialogue. As a baseline for subsequent analysis, this framework can inform AI improvement by studying to acknowledge when anthropomorphic traits happen and their impact on customers. Future improvement could make evaluation strategies extra exact, improve the robustness of metrics, and formalize evaluation, resulting in extra clear and morally sound AI techniques.
Try the Paper. All credit score for this analysis goes to the researchers of this challenge. Additionally, be happy to comply with us on Twitter and don’t neglect to affix our 75k+ ML SubReddit.
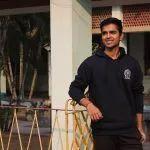
Divyesh is a consulting intern at Marktechpost. He’s pursuing a BTech in Agricultural and Meals Engineering from the Indian Institute of Expertise, Kharagpur. He’s a Information Science and Machine studying fanatic who needs to combine these main applied sciences into the agricultural area and resolve challenges.