On this tutorial, we’ll construct a completely practical Retrieval-Augmented Era (RAG) pipeline utilizing open-source instruments that run seamlessly on Google Colab. First, we’ll look into how one can arrange Ollama and use fashions by Colab. Integrating the DeepSeek-R1 1.5B massive language mannequin served by Ollama, the modular orchestration of LangChain, and the high-performance ChromaDB vector retailer permits customers to question real-time info extracted from uploaded PDFs. With a mix of native language mannequin reasoning and retrieval of factual information from PDF paperwork, the pipeline demonstrates a strong, non-public, and cost-effective different.
!pip set up colab-xterm
%load_ext colabxterm
We use the colab-xterm extension to allow terminal entry instantly inside the Colab atmosphere. By putting in it with !pip set up collab and loading it through %load_ext colabxterm, customers can open an interactive terminal window inside Colab, making it simpler to run instructions like llama serve or monitor native processes.
The %xterm magic command is used after loading the collab extension to launch an interactive terminal window inside the Colab pocket book interface. This enables customers to execute shell instructions in actual time, identical to an everyday terminal, making it particularly helpful for working background providers like llama serve, managing recordsdata, or debugging system-level operations with out leaving the pocket book.
Right here, we set up ollama utilizing curl https://ollama.ai/set up.sh | sh.
Then, we begin the ollama utilizing ollama serve.
Finally, we obtain the DeepSeek-R1:1.5B by ollama domestically that may be utilized for constructing the RAG pipeline.
!pip set up langchain langchain-community sentence-transformers chromadb faiss-cpu
To arrange the core elements of the RAG pipeline, we set up important libraries, together with langchain, langchain-community, sentence-transformers, chromadb, and faiss-cpu. These packages allow doc processing, embedding, vector storage, and retrieval functionalities required to construct an environment friendly and modular native RAG system.
from langchain_community.document_loaders import PyPDFLoader
from langchain.text_splitter import RecursiveCharacterTextSplitter
from langchain_community.vectorstores import Chroma
from langchain_community.embeddings import HuggingFaceEmbeddings
from langchain_community.llms import Ollama
from langchain.chains import RetrievalQA
from google.colab import recordsdata
import os
from langchain_core.prompts import ChatPromptTemplate
from langchain_ollama.llms import OllamaLLM
We import key modules from the langchain-community and langchain-ollama libraries to deal with PDF loading, textual content splitting, embedding technology, vector storage with Chroma, and LLM integration through Ollama. It additionally contains Colab’s file add utility and immediate templates, enabling a seamless movement from doc ingestion to question answering utilizing a domestically hosted mannequin.
print("Please add your PDF file...")
uploaded = recordsdata.add()
file_path = checklist(uploaded.keys())[0]
print(f"File '{file_path}' efficiently uploaded.")
if not file_path.decrease().endswith('.pdf'):
print("Warning: Uploaded file is just not a PDF. This may occasionally trigger points.")
To permit customers so as to add their data sources, we immediate for a PDF add utilizing google.colab.recordsdata.add(). It verifies the uploaded file kind and supplies suggestions, making certain that solely PDFs are processed for additional embedding and retrieval.
!pip set up pypdf
import pypdf
loader = PyPDFLoader(file_path)
paperwork = loader.load()
print(f"Efficiently loaded {len(paperwork)} pages from PDF")
To extract content material from the uploaded PDF, we set up the pypdf library and use PyPDFLoader from LangChain to load the doc. This course of converts every web page of the PDF right into a structured format, enabling downstream duties like textual content splitting and embedding.
text_splitter = RecursiveCharacterTextSplitter(
chunk_size=1000,
chunk_overlap=200
)
chunks = text_splitter.split_documents(paperwork)
print(f"Break up paperwork into {len(chunks)} chunks")
The loaded PDF is cut up into manageable chunks utilizing RecursiveCharacterTextSplitter, with every chunk sized at 1000 characters and a 200-character overlap. This ensures higher context retention throughout chunks, which improves the relevance of retrieved passages throughout query answering.
embeddings = HuggingFaceEmbeddings(
model_name="all-MiniLM-L6-v2",
model_kwargs={'machine': 'cpu'}
)
persist_directory = "./chroma_db"
vectorstore = Chroma.from_documents(
paperwork=chunks,
embedding=embeddings,
persist_directory=persist_directory
)
vectorstore.persist()
print(f"Vector retailer created and endured to {persist_directory}")
The textual content chunks are embedded utilizing the all-MiniLM-L6-v2 mannequin from sentence-transformers, working on CPU to allow semantic search. These embeddings are then saved in a persistent ChromaDB vector retailer, permitting environment friendly similarity-based retrieval throughout periods.
llm = OllamaLLM(mannequin="deepseek-r1:1.5b")
retriever = vectorstore.as_retriever(
search_type="similarity",
search_kwargs={"ok": 3}
)
qa_chain = RetrievalQA.from_chain_type(
llm=llm,
chain_type="stuff",
retriever=retriever,
return_source_documents=True
)
print("RAG pipeline created efficiently!")
The RAG pipeline is finalized by connecting the native DeepSeek-R1 mannequin (through OllamaLLM) with the Chroma-based retriever. Utilizing LangChain’s RetrievalQA chain with a “stuff” technique, the mannequin retrieves the highest 3 most related chunks to a question and generates context-aware solutions, finishing the native RAG setup.
def query_rag(query):
consequence = qa_chain({"question": query})
print("nQuestion:", query)
print("nAnswer:", consequence["result"])
print("nSources:")
for i, doc in enumerate(consequence["source_documents"]):
print(f"Supply {i+1}:n{doc.page_content[:200]}...n")
return consequence
query = "What's the principal matter of this doc?"
consequence = query_rag(query)
To check the RAG pipeline, a query_rag perform takes a consumer query, retrieves related context utilizing the retriever, and generates a solution utilizing the LLM. It additionally shows the highest supply paperwork, offering transparency and traceability for the mannequin’s response.
In conclusion, this tutorial combines ollama, the retrieval energy of ChromaDB, the orchestration capabilities of LangChain, and the reasoning talents of DeepSeek-R1 through Ollama. It showcased constructing a light-weight but highly effective RAG system that runs effectively on Google Colab’s free tier. The answer allows customers to ask questions grounded in up-to-date content material from uploaded paperwork, with solutions generated by a neighborhood LLM. This structure supplies a basis for constructing scalable, customizable, and privacy-friendly AI assistants with out incurring cloud prices or compromising efficiency.
Right here is the Colab Notebook. Additionally, don’t overlook to observe us on Twitter and be a part of our Telegram Channel and LinkedIn Group. Don’t Overlook to affix our 85k+ ML SubReddit.
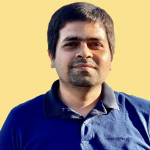
Asif Razzaq is the CEO of Marktechpost Media Inc.. As a visionary entrepreneur and engineer, Asif is dedicated to harnessing the potential of Synthetic Intelligence for social good. His most up-to-date endeavor is the launch of an Synthetic Intelligence Media Platform, Marktechpost, which stands out for its in-depth protection of machine studying and deep studying information that’s each technically sound and simply comprehensible by a large viewers. The platform boasts of over 2 million month-to-month views, illustrating its recognition amongst audiences.