Course of-supervised reward fashions (PRMs) supply fine-grained, step-wise suggestions on mannequin responses, aiding in deciding on efficient reasoning paths for advanced duties. Not like output reward fashions (ORMs), which consider responses based mostly on last outputs, PRMs present detailed assessments at every step, making them significantly useful for reasoning-intensive functions. Whereas PRMs have been extensively studied in language duties, their utility in multimodal settings stays largely unexplored. Most vision-language reward fashions nonetheless depend on the ORM strategy, highlighting the necessity for additional analysis into how PRMs can improve multimodal studying and reasoning.
Present reward benchmarks primarily give attention to text-based fashions, with some particularly designed for PRMs. Within the vision-language area, analysis strategies typically assess broad mannequin capabilities, together with data, reasoning, equity, and security. VL-RewardBench is the primary benchmark incorporating reinforcement studying desire information to refine knowledge-intensive vision-language duties. Moreover, multimodal RewardBench expands analysis standards past customary visible query answering (VQA) duties, protecting six key areas—correctness, desire, data, reasoning, security, and VQA—via professional annotations. These benchmarks present a basis for growing more practical reward fashions for multimodal studying.
Researchers from UC Santa Cruz, UT Dallas, and Amazon Analysis benchmarked VLLMs as ORMs and PRMs throughout a number of duties, revealing that neither persistently outperforms the opposite. To handle analysis gaps, they launched VILBENCH, a benchmark requiring step-wise reward suggestions, the place GPT-4o with Chain-of-Thought achieved solely 27.3% accuracy. Moreover, they collected 73.6K vision-language reward samples utilizing an enhanced tree-search algorithm, coaching a 3B PRM that improved analysis accuracy by 3.3%. Their examine gives insights into vision-language reward modeling and highlights challenges in multimodal step-wise analysis.
VLLMs are more and more efficient throughout numerous duties, significantly when evaluated for test-time scaling. Seven fashions had been benchmarked utilizing the LLM-as-a-judge strategy to research their step-wise critique talents on 5 vision-language datasets. A Greatest-of-N (BoN) setting was used, the place VLLMs scored responses generated by GPT-4o. Key findings reveal that ORMs outperform PRMs usually apart from real-world duties. Moreover, stronger VLLMs don’t all the time excel as reward fashions, and a hybrid strategy between ORM and PRM is perfect. Furthermore, VLLMs profit from text-heavy duties greater than visible ones, underscoring the necessity for specialised vision-language reward fashions.
To evaluate ViLPRM’s effectiveness, experiments had been performed on VILBENCH utilizing totally different RMs and resolution samplers. The examine in contrast efficiency throughout a number of VLLMs, together with Qwen2.5-VL-3B, InternVL-2.5-8B, GPT-4o, and o1. Outcomes present that PRMs typically outperform ORMs, bettering accuracy by 1.4%, although o1’s responses confirmed minimal distinction attributable to restricted element. ViLPRM surpassed different PRMs, together with URSA, by 0.9%, demonstrating superior consistency in response choice. Moreover, findings recommend that current VLLMs aren’t strong sufficient as reward fashions, highlighting the necessity for specialised vision-language PRMs that carry out nicely past math reasoning duties.
In conclusion, Imaginative and prescient-language PRMs carry out nicely when reasoning steps are segmented, as seen in structured duties like arithmetic. Nonetheless, in capabilities with unclear step divisions, PRMs can scale back accuracy, significantly in visual-dominant circumstances. Prioritizing key steps quite than treating all equally improves efficiency. Moreover, present multimodal reward fashions battle with generalization, as PRMs skilled on particular domains typically fail in others. Enhancing coaching by incorporating various information sources and adaptive reward mechanisms is essential. The introduction of ViLReward-73K improves PRM accuracy by 3.3%, however additional developments in step segmentation and analysis frameworks are wanted for strong multimodal fashions.
Check out the Paper. All credit score for this analysis goes to the researchers of this venture. Additionally, be at liberty to comply with us on Twitter and don’t neglect to affix our 85k+ ML SubReddit.
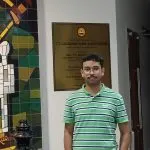
Sana Hassan, a consulting intern at Marktechpost and dual-degree scholar at IIT Madras, is captivated with making use of know-how and AI to handle real-world challenges. With a eager curiosity in fixing sensible issues, he brings a contemporary perspective to the intersection of AI and real-life options.