Proteins, important macromolecules for organic processes like metabolism and immune response, observe the sequence-structure-function paradigm, the place amino acid sequences decide 3D buildings and capabilities. Computational protein science AIms to decode this relationship and design proteins with desired properties. Conventional AI fashions have achieved important success in particular protein modeling duties, reminiscent of construction prediction and design. Nevertheless, these fashions face challenges in understanding the “grammar” and “semantics” of protein sequences and lack generalization throughout duties. Just lately, protein Language Fashions (pLMs) leveraging LLM methods have emerged, enabling developments in protein understanding, perform prediction, and design.
Researchers from establishments like The Hong Kong Polytechnic College, Michigan State College, and Mohamed bin Zayed College of Synthetic Intelligence have superior computational protein science by integrating LLMs to develop pLMs. These fashions successfully seize protein data and deal with sequence-structure-function reasoning issues. This survey systematically categorizes pLMs into sequence-based, structure- and function-enhanced, and multimodal fashions, exploring their purposes in protein construction prediction, perform prediction, and design. It highlights pLMs’ influence on antibody design, enzyme engineering, and drug discovery whereas discussing challenges and future instructions, offering insights for AI and biology researchers on this rising area.
Protein construction prediction is a crucial problem in computational biology as a result of complexity of experimental methods like X-ray crystallography and NMR. Latest developments like AlphaFold2 and RoseTTAFold have considerably improved construction prediction by incorporating evolutionary and geometric constraints. Nevertheless, these strategies nonetheless face challenges, particularly with orphan proteins missing homologous sequences. To handle these points, single-sequence prediction strategies, like ESMFold, use pLMs to foretell protein buildings with out counting on a number of sequence alignments (MSAs). These strategies provide sooner and extra common predictions, notably for proteins with no homology, although there’s nonetheless room for enchancment in accuracy.
pLMs have considerably impacted computational and experimental protein science, notably in purposes like antibody design, enzyme design, and drug discovery. In antibody design, pLMs can suggest antibody sequences that particularly bind to focus on antigens, providing a extra managed and cost-effective different to conventional animal-based strategies. These fashions, like PALMH3, have efficiently designed antibodies focusing on varied SARS-CoV-2 variants, demonstrating improved neutralization and affinity. Equally, pLMs play a key function in enzyme design by optimizing wild-type enzymes for enhanced stability and new catalytic capabilities. For instance, InstructPLM has been used to revamp enzymes like PETase and L-MDH, bettering their effectivity in comparison with the wild-type.
In drug discovery, pLMs assist predict interactions between medicine and goal proteins, accelerating the screening of potential drug candidates. Fashions like TransDTI can classify drug-target interactions, aiding in figuring out promising compounds for illnesses. Moreover, ConPLex leverages contrastive studying to foretell kinase-drug interactions, efficiently confirming a number of high-affinity binding interactions. These advances in pLM purposes streamline the drug discovery course of and contribute to growing simpler therapies with higher effectivity and security profiles.
In conclusion, the research offers an in-depth take a look at the function of LLMs in protein science, protecting each foundational ideas and up to date developments. It discusses the organic foundation of protein modeling, the categorization of pLMs based mostly on their means to know sequences, buildings, and practical info, and their purposes in protein construction prediction, perform prediction, and design. The overview additionally highlights pLMs’ potential in sensible fields like antibody design, enzyme engineering, and drug discovery. Lastly, it outlines promising future instructions on this quickly advancing area, emphasizing the transformative influence of AI on computational protein science.
Take a look at the Paper. All credit score for this analysis goes to the researchers of this mission. Additionally, don’t overlook to observe us on Twitter and be part of our Telegram Channel and LinkedIn Group. Don’t Overlook to hitch our 70k+ ML SubReddit.
🚨 [Recommended Read] Nebius AI Studio expands with vision models, new language models, embeddings and LoRA (Promoted)
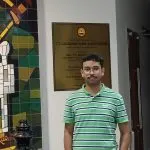
Sana Hassan, a consulting intern at Marktechpost and dual-degree pupil at IIT Madras, is captivated with making use of know-how and AI to deal with real-world challenges. With a eager curiosity in fixing sensible issues, he brings a contemporary perspective to the intersection of AI and real-life options.