Giant language fashions have made important strides in understanding and producing human-like textual content. But, with regards to complicated reasoning duties—particularly people who require multi-step calculations or logical evaluation—they usually battle. Conventional chain-of-thought (CoT) approaches assist by breaking down issues into intermediate steps, however they rely closely on the mannequin’s inside reasoning. This inside dependency can generally result in errors, notably with intricate computations or when a number of reasoning steps are wanted. In such instances, minor errors could accumulate, leading to outcomes that aren’t as exact as anticipated. The necessity for a technique that may confirm and modify its personal reasoning is obvious, particularly in duties like scientific evaluation or competition-level arithmetic.
Researchers at Alibaba have proposed a brand new AI instrument known as START, which stands for Self-Taught Reasoner with Instruments. Somewhat than relying solely on inside logic, START integrates an exterior Python interpreter to help with reasoning duties. The mannequin is constructed on a fine-tuned model of the QwQ-32B mannequin and employs a two-fold technique to enhance its problem-solving abilities. First, it makes use of a technique known as Trace-infer. Right here, the mannequin is inspired to incorporate prompts like “Wait, perhaps utilizing Python right here is a good suggestion,” which sign that it ought to carry out computations or self-check its work utilizing exterior instruments. Second, the mannequin undergoes a fine-tuning course of generally known as Trace Rejection Sampling Effective-Tuning (Trace-RFT). This course of refines the mannequin’s reasoning by filtering and modifying its output based mostly on how successfully it could invoke exterior instruments. The result’s a mannequin that’s not solely able to producing a logical chain of thought but in addition of verifying its steps by means of exterior computation.

Technical Insights and Advantages
At its core, START is an evolution of the chain-of-thought method. Its two-stage coaching course of is designed to assist the mannequin use exterior instruments as a pure extension of its reasoning course of. Within the first stage, Trace-infer permits the mannequin to combine cues that immediate instrument utilization. These hints are strategically inserted at factors the place the mannequin may be reconsidering its method, usually after transitional phrases like “Alternatively” or “Wait.” This encourages the mannequin to confirm its reasoning with Python code, resulting in self-correction when vital.
Within the second stage, Trace-RFT takes the output generated with these hints and refines it. By scoring and filtering the reasoning steps, the mannequin learns to higher resolve when and the way to invoke exterior instruments. The refined dataset from this course of is then used to fine-tune the mannequin additional, leading to a model of QwQ-32B that we now name START. The combination of exterior computation is a considerate addition that helps decrease errors, guaranteeing that the mannequin’s reasoning is each coherent and extra dependable.

Empirical Findings and Insights
The researchers evaluated START on a spread of duties, together with graduate-level science questions, difficult math issues, and programming duties. Throughout these domains, START confirmed notable enhancements over its base mannequin. For instance, on a set of PhD-level science questions, the mannequin achieved an accuracy of 63.6%, which is a modest but significant enchancment over the unique mannequin’s efficiency. On math benchmarks—starting from highschool stage to competitors issues—the accuracy enhancements have been equally encouraging. These outcomes recommend that the power to include exterior verification can result in higher problem-solving, particularly in duties the place precision is essential.
In programming challenges, START’s method allowed it to generate and take a look at code snippets, resulting in the next price of right options in comparison with fashions that rely solely on inside reasoning. Total, the examine signifies that the combination of instrument utilization inside the reasoning course of will help fashions produce extra correct and verifiable outcomes.

Concluding Ideas
The event of START gives a considerate step ahead in addressing the inherent challenges of complicated reasoning in giant language fashions. By combining inside chain-of-thought reasoning with exterior instrument integration, the mannequin gives a sensible answer to among the persistent points in computational and logical duties. The method is each easy and chic: encouraging the mannequin to self-check its work utilizing an exterior Python interpreter after which fine-tuning it based mostly on this skill results in improved efficiency throughout numerous benchmarks.
This work is a promising instance of how incremental refinements—on this case, using strategic hints and exterior computation—can considerably improve the reliability of reasoning in language fashions. It demonstrates that by thoughtfully integrating exterior instruments, we are able to information fashions towards extra correct and dependable outcomes, particularly in areas the place exact computation and logical rigor are important. The work behind START is an encouraging transfer towards fashions that aren’t solely extra succesful but in addition extra reflective and self-correcting of their method to problem-solving.
Check out the Paper. All credit score for this analysis goes to the researchers of this mission. Additionally, be at liberty to comply with us on Twitter and don’t neglect to affix our 80k+ ML SubReddit.
🚨 Really useful Learn- LG AI Analysis Releases NEXUS: An Superior System Integrating Agent AI System and Information Compliance Requirements to Deal with Authorized Issues in AI Datasets
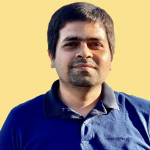
Asif Razzaq is the CEO of Marktechpost Media Inc.. As a visionary entrepreneur and engineer, Asif is dedicated to harnessing the potential of Synthetic Intelligence for social good. His most up-to-date endeavor is the launch of an Synthetic Intelligence Media Platform, Marktechpost, which stands out for its in-depth protection of machine studying and deep studying information that’s each technically sound and simply comprehensible by a large viewers. The platform boasts of over 2 million month-to-month views, illustrating its reputation amongst audiences.