Imaginative and prescient-language fashions (VLMs) face a important problem in attaining strong generalization past their coaching knowledge whereas sustaining computational assets and value effectivity. Approaches, resembling chain-of-thought supervised fine-tuning (CoT-SFT), usually result in overfitting, the place fashions carry out nicely on seen knowledge however wrestle with new, unseen eventualities. This limitation reduces their effectiveness in purposes that demand adaptability, resembling autonomous programs, medical imaging, and visible reasoning duties. Additionally, the prevailing assumption is that rising mannequin dimension is the important thing to improved efficiency. The necessity for a extra environment friendly coaching paradigm that enhances generalization, minimizes overfitting and reduces computational prices has develop into essential for advancing VLMs.
Deep Agent released R1-V to resolve a few of the above considerations. This novel reinforcement studying strategy enhances the generalization capability of VLMs whereas being cost-effective. This strategy demonstrates how reinforcement studying with verifiable rewards (RLVR) can outperform conventional CoT-SFT in effectiveness and robustness when coping with out-of-distribution (OOD) knowledge.
The primary goal of the R1-V strategy is to reinforce VLMs’ capability to generalize past their coaching datasets. R1-V tackles this concern by using reinforcement studying methods that information the mannequin to be taught generalizable abilities fairly than memorizing coaching examples. Specifically, it focuses on educating VLMs to develop strong visible counting skills, a necessary ability in lots of AI purposes, together with picture recognition, autonomous programs, and visible reasoning.
A serious spotlight of R1-V is its coaching effectivity. Regardless of using a comparatively small mannequin with solely 2 billion parameters, R1-V performs higher than a considerably bigger 72 billion parameter mannequin in OOD checks. This demonstrates that mannequin dimension is just not the only real determinant of efficiency; the coaching methodology and reinforcement studying methods are essential in enhancing a mannequin’s capabilities.
R1-V was educated on eight A100 GPUs for half-hour, with a complete computational price of solely $2.62. This cost-effectiveness makes it a gorgeous various for researchers and builders who want to obtain excessive efficiency with out in depth computational assets. R1-V additionally stands out attributable to its reliance on a curated coaching dataset. The mannequin was educated utilizing CLEVR-70k and R1-Distilled Visual Reasoning datasets, particularly designed to encourage visible reasoning and strong decision-making. Utilizing these datasets ensures that the mannequin develops a deep understanding of visible relationships and logical reasoning fairly than merely studying to acknowledge patterns from a given dataset.
In conclusion, the event of R1-V helps open-source AI analysis by making its code, mannequin weights, datasets, and coaching scripts publicly accessible. This enables the AI analysis group to refine and enhance vision-language modeling. R1-V’s reinforcement studying strategy permits fast studying of patterns and buildings in knowledge. It results in excessive efficiency with minimal computational price. This challenges the idea that in depth coaching and big datasets are mandatory for state-of-the-art AI efficiency. As a substitute, environment friendly coaching methodologies can scale back computational calls for whereas sustaining or surpassing conventional outcomes.
Take a look at the GitHub Page. All credit score for this analysis goes to the researchers of this mission. Additionally, don’t overlook to observe us on Twitter and be part of our Telegram Channel and LinkedIn Group. Don’t Overlook to hitch our 75k+ ML SubReddit.
🚨 Marktechpost is inviting AI Corporations/Startups/Teams to associate for its upcoming AI Magazines on ‘Open Supply AI in Manufacturing’ and ‘Agentic AI’.
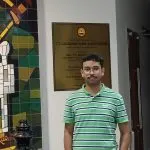
Sana Hassan, a consulting intern at Marktechpost and dual-degree pupil at IIT Madras, is enthusiastic about making use of know-how and AI to handle real-world challenges. With a eager curiosity in fixing sensible issues, he brings a contemporary perspective to the intersection of AI and real-life options.