Imaginative and prescient-Language Fashions (VLMs) permit machines to grasp and purpose in regards to the visible world via pure language. These fashions have functions in picture captioning, visible query answering, and multimodal reasoning. Nonetheless, most fashions are designed and skilled predominantly for high-resource languages, leaving substantial gaps in accessibility and usefulness for audio system of low-resource languages. This hole highlights the significance of growing multilingual techniques that cater to a world viewers whereas sustaining excessive efficiency throughout various linguistic and cultural contexts. Nonetheless, a priority in growing multilingual VLMs lies within the availability and high quality of multilingual datasets.
Even when there are datasets, they’ve these limitations:
- Present pretraining datasets, resembling COCO, Visible Genome, and LAION, are overwhelmingly targeted on English, limiting their generalization skill throughout languages and cultures.
- Many datasets additionally include poisonous or biased content material, perpetuating stereotypes and undermining the moral deployment of AI techniques.
The restricted illustration of various languages, mixed with the presence of culturally insensitive materials, hampers the efficiency of VLMs in underrepresented areas and raises considerations about equity and inclusivity.
Researchers have turned to numerous strategies of dataset enlargement and high quality enchancment to handle these limitations. For instance, datasets like Multi30k and Crossmodal-3600 have tried to supply multilingual assist however have to be expanded in scale and variety. Semi-automated translations of image-text datasets have been used to increase language protection in fashions resembling PALO and X-LLaVA. Nonetheless, these efforts typically end in uneven distributions throughout languages and fail to handle the toxicity current within the authentic knowledge. The shortage of systematic approaches to filtering dangerous content material additional worsens the problem.
A staff of researchers from Cisco Meraki, Cohere For AI Neighborhood, Indiana College Bloomington, Imperial School London, Georgia Institute of Know-how, The Alan Turing Institute, Bangladesh College of Engineering and Know-how, College of Pennsylvania, IIT Bombay, TU Darmstadt, Articul8 AI, Capital One, IIT Dhanbad, and MBZUAI launched Maya, an 8B parameters open-source multilingual multimodal vision-language mannequin that goals to beat present dataset high quality and toxicity limitations. The mannequin leverages a brand new pretraining dataset containing 558,000 image-text pairs distributed equally throughout eight languages: English, Chinese language, French, Spanish, Russian, Hindi, Japanese, and Arabic. This dataset underwent rigorous toxicity filtering, with over 7,531 poisonous photographs and captions eliminated utilizing instruments like LLaVAGuard and Poisonous-BERT. Maya’s growth additionally targeted on balancing knowledge distribution to forestall biases.
Maya’s structure is constructed on the LLaVA framework and incorporates superior methods for image-text alignment and multilingual adaptation. The mannequin employs SigLIP, a imaginative and prescient encoder able to dealing with variable enter dimensions, and Aya-23, a multilingual language mannequin skilled throughout 23 languages. A two-layer projection matrix bridges picture options to language options, optimizing efficiency whereas sustaining computational effectivity. Pretraining was performed on 8xH100 GPUs with a world batch dimension of 256; instruction fine-tuning utilized the PALO 150K dataset. This coaching course of was designed to make sure high-quality outputs, with pretraining taking roughly 20 hours and fine-tuning requiring 48 hours.
Efficiency-wise, on multilingual benchmarks resembling LLaVA-Bench-In-The-Wild, Maya outperformed similar-size fashions like LLaVA-7B and PALO-7B in 5 out of eight languages, together with notable success in Arabic resulting from its sturdy translation and dataset design. Throughout English-only benchmarks, Maya maintained aggressive accuracy, with marginal beneficial properties noticed in duties like textual content translation and numerical calculation for the toxicity-free variant. Nonetheless, some advanced reasoning duties confirmed slight efficiency declines, indicating that eradicating various, probably poisonous content material might impression sure capabilities.
Some key takeaways and highlights from the Maya mannequin analysis are summarized beneath:
- Maya’s pretraining dataset consists of 558,000 image-text pairs expanded to 4.4 million samples throughout eight languages. Rigorous toxicity filtering eliminated 7,531 poisonous components, making certain cleaner knowledge.
- The mannequin helps eight languages, attaining balanced knowledge distribution and cultural inclusivity via optimized translation and pretraining methods.
- SigLIP for imaginative and prescient encoding and Aya-23 for multilingual language modeling allow high-quality image-text alignment and cross-linguistic comprehension.
- Maya outperformed comparable fashions in 5 languages and matched bigger fashions in a number of benchmarks.
- Maya units a precedent for moral and truthful AI practices by addressing toxicity and biases.
In conclusion, by introducing Maya, the analysis addresses restricted multilingual and culturally delicate datasets in VLMs. This mannequin combines an modern dataset of 558,000 image-text pairs throughout eight languages with rigorous toxicity filtering and balanced illustration to make sure inclusivity and moral deployment. Leveraging superior structure and multilingual adaptation methods, Maya outperforms similar-size fashions in a number of languages, setting a brand new commonplace for multilingual AI.
Try the Paper and Model on Hugging Face. All credit score for this analysis goes to the researchers of this challenge. Additionally, don’t neglect to observe us on Twitter and be a part of our Telegram Channel and LinkedIn Group. Don’t Neglect to affix our 60k+ ML SubReddit.
🚨 [Must Subscribe]: Subscribe to our newsletter to get trending AI research and dev updates
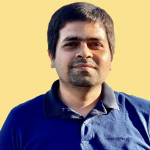
Asif Razzaq is the CEO of Marktechpost Media Inc.. As a visionary entrepreneur and engineer, Asif is dedicated to harnessing the potential of Synthetic Intelligence for social good. His most up-to-date endeavor is the launch of an Synthetic Intelligence Media Platform, Marktechpost, which stands out for its in-depth protection of machine studying and deep studying information that’s each technically sound and simply comprehensible by a large viewers. The platform boasts of over 2 million month-to-month views, illustrating its reputation amongst audiences.