Multimodal AI permits machines to course of and motive throughout varied enter codecs, resembling pictures, textual content, movies, and sophisticated paperwork. This area has seen elevated curiosity as conventional language fashions, whereas highly effective, are insufficient when confronted with visible knowledge or when contextual interpretation spans throughout a number of enter sorts. The true world is inherently multimodal, so programs aiming to help in real-time duties, analyzing consumer interfaces, understanding tutorial supplies, or decoding advanced scenes require intelligence that features past textual reasoning. Newer fashions at the moment are being developed to concurrently decode language and imaginative and prescient cues to method duties with improved contextual consciousness, reasoning depth, and flexibility to totally different knowledge enter kinds.
A limitation in multimodal programs at present lies of their lack of ability to course of lengthy contexts effectively and to generalize throughout high-resolution or numerous enter buildings with out compromising efficiency. Many open-source fashions restrict the enter to some thousand tokens or demand extreme computational sources to keep up efficiency at scale. These constraints end in fashions that will carry out nicely on commonplace benchmarks however wrestle with real-world functions that contain advanced, multi-image inputs, prolonged dialogues, or tutorial duties like OCR-based doc evaluation and mathematical problem-solving. There’s additionally a spot in reasoning skill, significantly long-horizon pondering, which prevents present programs from dealing with duties that require step-by-step logic or deep contextual alignment between totally different knowledge modalities.
Earlier instruments have tried to deal with these challenges however typically fell brief in scalability or flexibility. The Qwen2.5-VL collection and Gemma-3 fashions, whereas notable for his or her dense architectures, lack built-in help for reasoning by way of longer chains of thought. Fashions like DeepSeek-VL2 and Aria adopted mixture-of-experts (MoE) methods however had fastened imaginative and prescient encoders that restricted their skill to adapt to varied resolutions and types of visible enter. Additionally, these fashions sometimes supported solely brief context home windows, 4K tokens in DeepSeek-VL2, and had restricted success in advanced OCR or multi-image situations. As such, most present programs did not stability low useful resource consumption with the power to deal with duties involving lengthy context and numerous visible knowledge.
Researchers at Moonshot AI launched Kimi-VL, a novel vision-language mannequin using an MoE structure. This technique prompts solely 2.8 billion parameters in its decoder, considerably lighter than many opponents whereas sustaining highly effective multimodal capabilities. The 2 launched fashions based mostly on this structure on Hugging Face are Kimi-VL-A3B-Thinking and Kimi-VL-A3B-Instruct. It incorporates a native-resolution visible encoder named MoonViT and helps context home windows of as much as 128K tokens. The mannequin has three built-in parts: the MoonViT encoder, an MLP projector for transitioning visible options to language embeddings, and the Moonlight MoE decoder. Researchers additional developed a complicated model, Kimi-VL-Considering, designed particularly for long-horizon reasoning duties by way of chain-of-thought supervised fine-tuning and reinforcement studying. Collectively, these fashions goal to redefine effectivity benchmarks in vision-language reasoning.
The architectural innovation in Kimi-VL lies in its adaptability and processing functionality. MoonViT processes high-resolution pictures of their unique type, eliminating the necessity for sub-image fragmentation. To make sure spatial consistency throughout various picture resolutions, the mannequin makes use of interpolated absolute positional embeddings mixed with two-dimensional rotary positional embeddings throughout each peak and width. These design decisions enable MoonViT to protect fine-grained element even in large-scale picture inputs. Outputs from the imaginative and prescient encoder are handed by way of a two-layer MLP that makes use of pixel shuffle operations to downsample spatial dimensions and convert options into LLM-compatible embeddings. On the language facet, the two.8B activated parameter MoE decoder helps 16B complete parameters and integrates seamlessly with visible representations, enabling extremely environment friendly coaching and inference throughout totally different enter sorts. The whole coaching course of used an enhanced Muon optimizer with weight decay and ZeRO-1-based reminiscence optimization for dealing with the massive parameter depend.
The coaching knowledge composition displays a concentrate on numerous multimodal studying. Beginning with 2.0T tokens for ViT coaching utilizing image-caption pairs, the workforce added one other 0.1T to align the encoder with the decoder. Joint pre-training consumed 1.4T tokens, adopted by 0.6T in cooldown and 0.3T in long-context activation, totaling 4.4T tokens. These levels included tutorial visible datasets, OCR samples, lengthy video knowledge, and artificial mathematical and code-based QA pairs. For long-context studying, the mannequin was progressively skilled to deal with sequences from 8K as much as 128K tokens, utilizing RoPE embeddings prolonged from a base frequency of fifty,000 to 800,000. This allowed the mannequin to keep up a token recall accuracy of 100% as much as 64K tokens, with a slight drop to 87.0% at 128K, nonetheless outperforming most options.
Kimi-VL demonstrated robust outcomes throughout a spread of benchmarks. On the LongVideoBench, it scored 64.5; on MMLongBench-Doc, it achieved 35.1; and on the InfoVQA benchmark, it led with 83.2. On ScreenSpot-Professional, which assessments understanding of UI screens, it scored 34.5. The Kimi-VL-Considering variant excelled in reasoning-intensive benchmarks like MMMU (61.7), MathVision (36.8), and MathVista (71.3). For agent duties resembling OSWorld, the mannequin matched or exceeded efficiency from bigger fashions like GPT-4o whereas activating considerably fewer parameters. Its compact design and powerful reasoning capabilities make it a number one candidate amongst open-source multimodal options.
Some Key Takeaways from the Analysis on Kimi-VL:
- Kimi-VL prompts solely 2.8B parameters throughout inference, making certain effectivity with out sacrificing functionality.
- MoonViT, its imaginative and prescient encoder, natively processes high-resolution pictures, bettering readability in duties like OCR and UI interpretation.
- The mannequin helps as much as 128K context tokens, attaining 100% recall as much as 64K and 87.0% accuracy at 128K on textual content/video duties.
- Kimi-VL-Considering scores 61.7 on MMMU, 36.8 on MathVision, and 71.3 on MathVista, outperforming many bigger VLMs.
- It scored 83.2 on InfoVQA and 34.5 on visible duties on ScreenSpot-Professional, showcasing its precision in perception-based evaluations.
- Complete pre-training concerned 4.4T tokens throughout textual content, video, doc, and artificial multimodal knowledge.
- Optimization was accomplished utilizing a custom-made Muon optimizer with memory-efficient methods like ZeRO-1.
- Joint coaching ensured seamless visible and language characteristic integration whereas preserving core language capabilities.
Take a look at Instruct Model and Reasoning Model. All credit score for this analysis goes to the researchers of this undertaking. Additionally, be happy to observe us on Twitter and don’t overlook to hitch our 85k+ ML SubReddit.
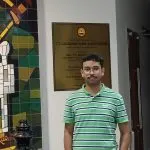
Sana Hassan, a consulting intern at Marktechpost and dual-degree scholar at IIT Madras, is keen about making use of know-how and AI to deal with real-world challenges. With a eager curiosity in fixing sensible issues, he brings a recent perspective to the intersection of AI and real-life options.