Massive language mdoels LLMs have proven outstanding efficiency throughout various textual content and multimodal duties. Nevertheless, many functions, comparable to doc and video understanding, in-context studying, and inference-time scaling, demand the flexibility to course of and cause over lengthy sequences of tokens. The restricted context window of LLMs poses a major problem in these conditions, as important data unfold over prolonged paperwork could also be neglected. Fashions usually miss important data when processing in depth paperwork or movies, falling outdoors their fixed-context home windows. This limitation creates a necessity for fashions that may effectively deal with ultra-long contexts with out sacrificing efficiency on customary duties.
Present context extension methods for long-context language fashions fall into three classes: precise consideration strategies, approximate consideration strategies, and approaches incorporating extra modules. Strategies like Place Interpolation, NTK-aware, Dynamic NTK, YaRN, and CLEX improve consideration mechanisms via redesigned place embeddings. Current developments embrace fashions like GPT-4o, Gemini, and Claude that assist in depth context home windows of tons of of hundreds of tokens, however their closed-source nature limits reproducibility. Open-source efforts like ProLong use NTK-aware scaling however require costly computation, whereas Gradient makes use of continued pretraining that comprises customary activity efficiency.
Researchers from UIUC and NVIDIA have proposed an environment friendly coaching recipe for constructing ultra-long context LLMs from aligned instruct fashions, pushing the boundaries of context lengths from 128K to 1M, 2M, and 4M tokens. The strategy makes use of environment friendly, continued pretraining methods to increase the context window whereas utilizing instruction tuning to take care of instruction-following and reasoning talents. Furthermore, their UltraLong-8B mannequin achieves state-of-the-art efficiency throughout various long-context benchmarks. Fashions skilled with this method preserve aggressive efficiency on customary benchmarks, displaying balanced enhancements for lengthy and quick context duties. The analysis supplies an in-depth evaluation of key design decisions, highlighting impacts of scaling methods and knowledge composition.
The proposed methodology consists of two key levels: continued pretraining and instruction tuning. Collectively, these levels allow the efficient processing of ultra-long inputs whereas sustaining sturdy efficiency throughout duties. A YaRN-based scaling method is adopted for context extension with mounted hyperparameters as α = 1 and β = 4 moderately than NTK-aware scaling methods. The size components are computed primarily based on the right track context size and make use of bigger scaling components for RoPE embeddings to accommodate prolonged sequences and mitigate efficiency degradation at most lengths. Researchers subsample high-quality SFT datasets spanning normal, arithmetic, and code domains for coaching knowledge and additional make the most of GPT-4o and GPT-4o-mini to refine responses and carry out rigorous knowledge decontamination.
The proposed fashions present superior long-context retrieval capabilities within the Needle in a Haystack passkey retrieval check. Baseline fashions like Llama-3-8B-Instruct-Gradient-1048k go the check, however Llama3.1-8B-Instruct and Llama-3-8B-ProLong-512k-Instruct present errors. In distinction, the UltraLong fashions obtain 100% accuracy throughout all enter lengths and depths, displaying sturdy retrieval functionality. The UltraLong achieves the best common scores on RULER for inputs as much as 512K and 1M tokens, the best F1 scores on LV-Eval inside 128K and 256K token lengths, and the perfect efficiency on InfiniteBench. Furthermore, the fashions preserve sturdy efficiency throughout normal, math, and code domains with common scores of 62.47, 61.06, and 60.95, exceeding the bottom mannequin’s 61.45.
This analysis paper introduces an environment friendly and systematic coaching recipe for ultra-long context language fashions, extending context home windows to 1M, 2M, and 4M tokens whereas sustaining aggressive efficiency on customary benchmarks. The method combines environment friendly continued pretraining with instruction tuning to reinforce long-context understanding and instruction-following capabilities. Nevertheless, this method focuses solely on SFT on instruction datasets throughout the instruction tuning stage with out exploring reinforcement studying or choice optimization. Additionally, it doesn’t tackle security alignment. Future analysis contains integrating security alignment mechanisms and exploring superior tuning methods, additional enhancing efficiency and trustworthiness.
Take a look at Paper and Model on Hugging Face. All credit score for this analysis goes to the researchers of this challenge. Additionally, be at liberty to comply with us on Twitter and don’t overlook to hitch our 85k+ ML SubReddit.
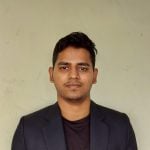
Sajjad Ansari is a remaining yr undergraduate from IIT Kharagpur. As a Tech fanatic, he delves into the sensible functions of AI with a give attention to understanding the affect of AI applied sciences and their real-world implications. He goals to articulate complicated AI ideas in a transparent and accessible method.