Mathematical reasoning has lengthy introduced a formidable problem for AI, demanding not solely an understanding of summary ideas but additionally the power to carry out multi-step logical deductions with precision. Conventional language fashions, whereas adept at producing fluent textual content, usually wrestle when tasked with fixing advanced mathematical issues that require each deep area data and structured reasoning. This hole has pushed analysis towards specialised architectures and coaching regimens designed to imbue fashions with sturdy mathematical capabilities. By specializing in focused datasets and fine-tuning methods, AI builders purpose to bridge the hole between pure language understanding and formal mathematical problem-solving.
NVIDIA has launched OpenMath-Nemotron-32B and OpenMath-Nemotron-14B-Kaggle, every meticulously engineered to excel in mathematical reasoning duties. Constructing on the success of the Qwen household of transformer fashions, these Nemotron variants make the most of large-scale fine-tuning on an in depth corpus of mathematical issues, collectively generally known as the OpenMathReasoning dataset. The design philosophy underlying each releases facilities on maximizing accuracy throughout aggressive benchmarks whereas sustaining sensible concerns for inference pace and useful resource effectivity. By providing a number of mannequin sizes and configurations, NVIDIA supplies researchers and practitioners with a versatile toolkit for integrating superior math capabilities into numerous functions.
OpenMath-Nemotron-32B represents the flagship of this collection, that includes 32.8 billion parameters and leveraging BF16 tensor operations for environment friendly {hardware} utilization. It’s constructed by fine-tuning Qwen2.5-32B on the OpenMathReasoning dataset, a curated assortment that emphasizes difficult issues drawn from mathematical Olympiads and standardized exams. This mannequin achieves state-of-the-art outcomes on a number of rigorous benchmarks, together with the American Invitational Arithmetic Examination (AIME) 2024 and 2025, the Harvard–MIT Arithmetic Match (HMMT) 2024-25, and the Harvard–London–Edinburgh Arithmetic Examination (HLE-Math) collection. In its tool-integrated reasoning (TIR) configuration, OpenMath-Nemotron-32B achieves a mean move@1 rating of 78.4 % on AIME24, with a majority-voting accuracy of 93.3 %, surpassing earlier top-performing fashions by notable margins.
To accommodate completely different inference eventualities, OpenMath-Nemotron-32B helps three distinct modes: chain-of-thought (CoT), tool-integrated reasoning (TIR), and generative resolution choice (GenSelect). In CoT mode, the mannequin generates intermediate reasoning steps earlier than presenting a ultimate reply, attaining a move@1 accuracy of 76.5% on AIME24. When augmented with GenSelect, which produces a number of candidate options and selects probably the most constant reply, the mannequin’s efficiency improves additional, attaining a outstanding 93.3% accuracy on the identical benchmark. These configurations allow customers to steadiness between rationalization richness and reply precision, catering to analysis environments that require transparency in addition to manufacturing settings that prioritize pace and reliability.
Complementing the 32 billion-parameter variant, NVIDIA has additionally launched OpenMath-Nemotron-14B-Kaggle, a 14.8 billion-parameter mannequin fine-tuned on a strategically chosen subset of the OpenMathReasoning dataset to optimize for aggressive efficiency. This model served because the cornerstone of NVIDIA’s first-place resolution within the AIMO-2 Kaggle competitors, a contest that centered on automated problem-solving strategies for superior mathematical challenges. By calibrating the coaching information to emphasise issues reflective of the competitors’s format and issue, the 14B-Kaggle mannequin demonstrated distinctive adaptability, outpacing rival approaches and securing the highest leaderboard place.
Efficiency benchmarks for OpenMath-Nemotron-14B-Kaggle mirror these of its bigger counterpart, with the mannequin attaining a move@1 accuracy of 73.7% on AIME24 in CoT mode and bettering to 86.7% underneath GenSelect protocols. On the AIME25 benchmark, it achieves a move fee of 57.9 % (majority at 64 of 73.3 %), and on HMMT-24-25, it attains 50.5 % (majority at 64 of 64.8 %). These figures spotlight the mannequin’s potential to ship high-quality options, even with a extra compact parameter footprint, making it well-suited for eventualities the place useful resource constraints or inference latency are crucial elements.
Each OpenMath-Nemotron fashions are accompanied by an open‐supply pipeline, enabling full reproducibility of knowledge era, coaching procedures, and analysis protocols. NVIDIA has built-in these workflows into its NeMo-Abilities framework, offering reference implementations for CoT, TIR, and GenSelect inference modes. With instance code snippets that reveal find out how to instantiate a transformer pipeline, configure dtype and machine mapping, and parse mannequin outputs, builders can quickly prototype functions that question these fashions for step-by-step options or streamlined ultimate solutions.
Beneath the hood, each fashions are optimized to run effectively on NVIDIA GPU architectures, starting from the Ampere to the Hopper microarchitectures, leveraging extremely tuned CUDA libraries and TensorRT optimizations. For manufacturing deployments, customers can serve fashions by way of Triton Inference Server, enabling low-latency, high-throughput integrations in internet providers or batch processing pipelines. The adoption of BF16 tensor codecs strikes a really perfect steadiness between numerical precision and reminiscence footprint, enabling these large-scale fashions to suit inside GPU reminiscence constraints whereas sustaining sturdy efficiency throughout numerous {hardware} platforms.
A number of Key Takeaways from the discharge of OpenMath-Nemotron-32B and OpenMath-Nemotron-14B-Kaggle embrace:
- NVIDIA’s OpenMath-Nemotron collection addresses the longstanding problem of equipping language fashions with sturdy mathematical reasoning by way of focused fine-tuning on the OpenMathReasoning dataset.
- The 32 B-parameter variant achieves state-of-the-art accuracy on benchmarks like AIME24/25 and HMMT, providing three inference modes (CoT, TIR, GenSelect) to steadiness rationalization richness and precision.
- The 14 B-parameter “Kaggle” mannequin, fine-tuned on a competition-focused subset, secured first place within the AIMO-2 Kaggle competitors whereas sustaining excessive move@1 scores, demonstrating effectivity in a smaller footprint.
- Each fashions are totally reproducible by way of an open-source pipeline built-in into NVIDIA’s NeMo-Abilities framework, with reference implementations for all inference modes.
- Optimized for NVIDIA GPUs (Ampere and Hopper), the fashions leverage BF16 tensor operations, CUDA libraries, TensorRT, and Triton Inference Server for low-latency, high-throughput deployments.
- Potential functions embrace AI-driven tutoring programs, educational competitors preparation instruments, and integration into scientific computing workflows requiring formal or symbolic reasoning.
- Future instructions could broaden to superior university-level arithmetic, multimodal inputs (e.g., handwritten equations), and tighter integration with symbolic computation engines to confirm and increase generated options.
Take a look at the OpenMath-Nemotron-32B and OpenMath-Nemotron-14B-Kaggle. Additionally, don’t neglect to observe us on Twitter and be part of our Telegram Channel and LinkedIn Group. Don’t Overlook to hitch our 90k+ ML SubReddit.
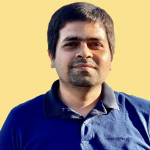
Asif Razzaq is the CEO of Marktechpost Media Inc.. As a visionary entrepreneur and engineer, Asif is dedicated to harnessing the potential of Synthetic Intelligence for social good. His most up-to-date endeavor is the launch of an Synthetic Intelligence Media Platform, Marktechpost, which stands out for its in-depth protection of machine studying and deep studying information that’s each technically sound and simply comprehensible by a large viewers. The platform boasts of over 2 million month-to-month views, illustrating its reputation amongst audiences.