A current paper from LG AI Analysis means that supposedly ‘open’ datasets used for coaching AI fashions could also be providing a false sense of safety – discovering that almost 4 out of 5 AI datasets labeled as ‘commercially usable’ really comprise hidden authorized dangers.
Such dangers vary from the inclusion of undisclosed copyrighted materials to restrictive licensing phrases buried deep in a dataset’s dependencies. If the paper’s findings are correct, firms counting on public datasets could have to rethink their present AI pipelines, or threat authorized publicity downstream.
The researchers suggest a radical and potentially controversial resolution: AI-based compliance brokers able to scanning and auditing dataset histories sooner and extra precisely than human legal professionals.
The paper states:
‘This paper advocates that the authorized threat of AI coaching datasets can’t be decided solely by reviewing surface-level license phrases; a radical, end-to-end evaluation of dataset redistribution is important for guaranteeing compliance.
‘Since such evaluation is past human capabilities as a result of its complexity and scale, AI brokers can bridge this hole by conducting it with better velocity and accuracy. With out automation, essential authorized dangers stay largely unexamined, jeopardizing moral AI growth and regulatory adherence.
‘We urge the AI analysis neighborhood to acknowledge end-to-end authorized evaluation as a basic requirement and to undertake AI-driven approaches because the viable path to scalable dataset compliance.’
Inspecting 2,852 fashionable datasets that appeared commercially usable primarily based on their particular person licenses, the researchers’ automated system discovered that solely 605 (round 21%) have been really legally protected for commercialization as soon as all their elements and dependencies have been traced
The new paper is titled Do Not Belief Licenses You See — Dataset Compliance Requires Large-Scale AI-Powered Lifecycle Tracing, and comes from eight researchers at LG AI Analysis.
Rights and Wrongs
The authors spotlight the challenges confronted by firms pushing ahead with AI growth in an more and more unsure authorized panorama – as the previous educational ‘honest use’ mindset round dataset coaching provides option to a fractured setting the place authorized protections are unclear and protected harbor is now not assured.
As one publication pointed out just lately, firms have gotten more and more defensive concerning the sources of their coaching information. Creator Adam Buick feedback*:
‘[While] OpenAI disclosed the principle sources of knowledge for GPT-3, the paper introducing GPT-4 revealed solely that the info on which the mannequin had been educated was a combination of ‘publicly accessible information (corresponding to web information) and information licensed from third-party suppliers’.
‘The motivations behind this transfer away from transparency haven’t been articulated in any specific element by AI builders, who in lots of instances have given no rationalization in any respect.
‘For its half, OpenAI justified its determination to not launch additional particulars concerning GPT-4 on the premise of considerations concerning ‘the aggressive panorama and the protection implications of large-scale fashions’, with no additional rationalization inside the report.’
Transparency generally is a disingenuous time period – or just a mistaken one; as an illustration, Adobe’s flagship Firefly generative mannequin, educated on inventory information that Adobe had the rights to take advantage of, supposedly supplied prospects reassurances concerning the legality of their use of the system. Later, some evidence emerged that the Firefly information pot had turn out to be ‘enriched’ with probably copyrighted information from different platforms.
As we mentioned earlier this week, there are rising initiatives designed to guarantee license compliance in datasets, together with one that can solely scrape YouTube movies with versatile Artistic Commons licenses.
The issue is that the licenses in themselves could also be inaccurate, or granted in error, as the brand new analysis appears to point.
Inspecting Open Supply Datasets
It’s tough to develop an analysis system such because the authors’ Nexus when the context is consistently shifting. Due to this fact the paper states that the NEXUS Knowledge Compliance framework system relies on ‘ numerous precedents and authorized grounds at this time limit’.
NEXUS makes use of an AI-driven agent known as AutoCompliance for automated information compliance. AutoCompliance is comprised of three key modules: a navigation module for internet exploration; a question-answering (QA) module for data extraction; and a scoring module for authorized threat evaluation.
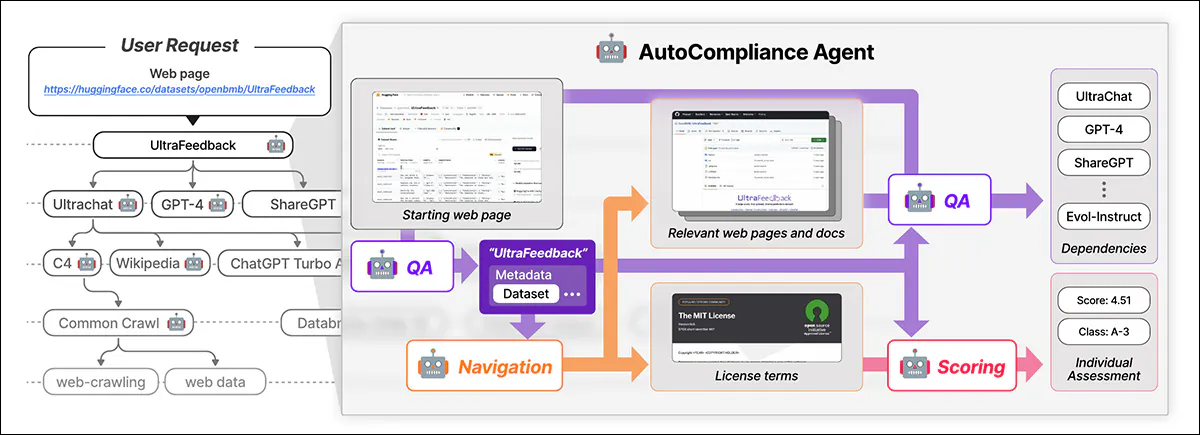
AutoCompliance begins with a user-provided webpage. The AI extracts key particulars, searches for associated assets, identifies license phrases and dependencies, and assigns a authorized threat rating. Supply: https://arxiv.org/pdf/2503.02784
These modules are powered by fine-tuned AI fashions, together with the EXAONE-3.5-32B-Instruct mannequin, educated on artificial and human-labeled information. AutoCompliance additionally makes use of a database for caching outcomes to reinforce effectivity.
AutoCompliance begins with a user-provided dataset URL and treats it as the foundation entity, trying to find its license phrases and dependencies, and recursively tracing linked datasets to construct a license dependency graph. As soon as all connections are mapped, it calculates compliance scores and assigns threat classifications.
The Knowledge Compliance framework outlined within the new work identifies numerous† entity varieties concerned within the information lifecycle, together with datasets, which kind the core enter for AI coaching; information processing software program and AI fashions, that are used to remodel and make the most of the info; and Platform Service Suppliers, which facilitate information dealing with.
The system holistically assesses authorized dangers by contemplating these numerous entities and their interdependencies, shifting past rote analysis of the datasets’ licenses to incorporate a broader ecosystem of the elements concerned in AI growth.
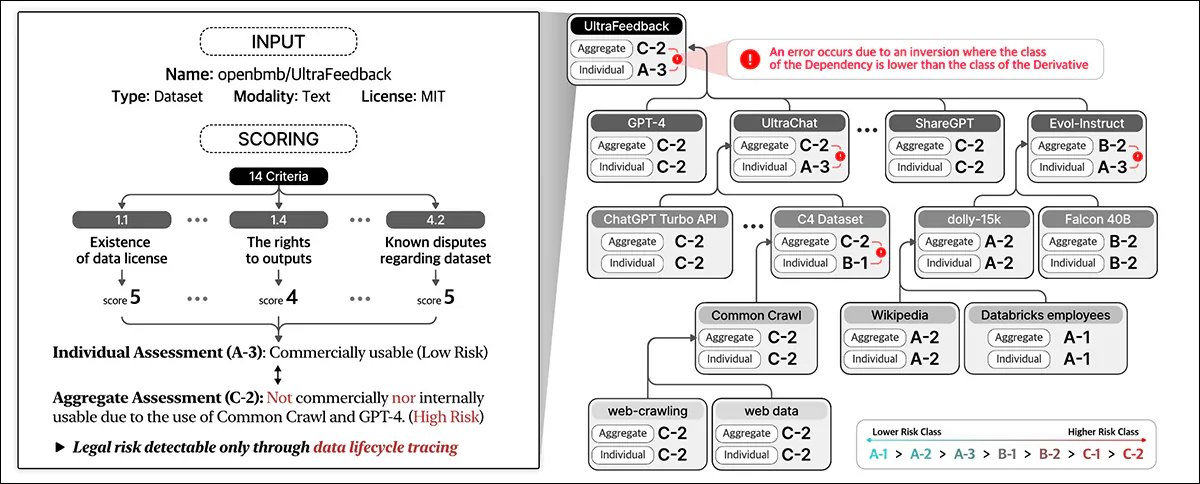
Knowledge Compliance assesses authorized threat throughout the complete information lifecycle. It assigns scores primarily based on dataset particulars and on 14 standards, classifying particular person entities and aggregating threat throughout dependencies.
Coaching and Metrics
The authors extracted the URLs of the highest 1,000 most-downloaded datasets at Hugging Face, randomly sub-sampling 216 objects to represent a check set.
The EXAONE mannequin was fine-tuned on the authors’ customized dataset, with the navigation module and question-answering module utilizing artificial information, and the scoring module utilizing human-labeled information.
Floor-truth labels have been created by 5 authorized specialists educated for at the least 31 hours in comparable duties. These human specialists manually recognized dependencies and license phrases for 216 check instances, then aggregated and refined their findings via dialogue.
With the educated, human-calibrated AutoCompliance system examined towards ChatGPT-4o and Perplexity Professional, notably extra dependencies have been found inside the license phrases:
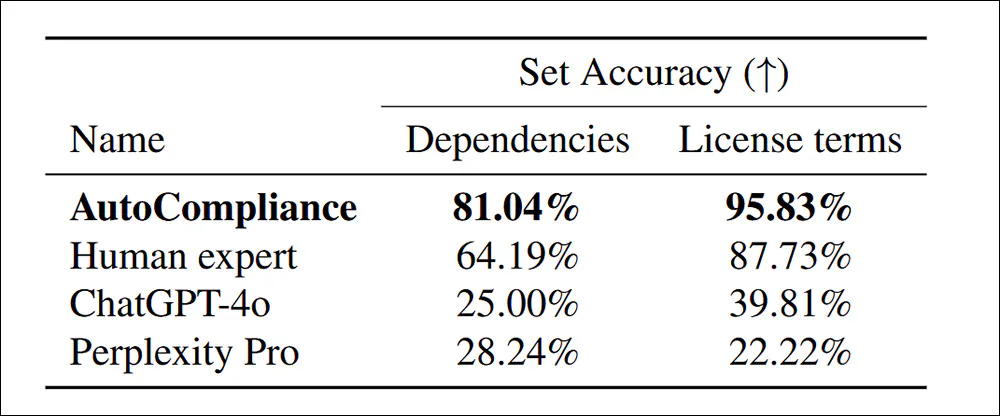
Accuracy in figuring out dependencies and license phrases for 216 analysis datasets.
The paper states:
‘The AutoCompliance considerably outperforms all different brokers and Human professional, reaching an accuracy of 81.04% and 95.83% in every process. In distinction, each ChatGPT-4o and Perplexity Professional present comparatively low accuracy for Supply and License duties, respectively.
‘These outcomes spotlight the superior efficiency of the AutoCompliance, demonstrating its efficacy in dealing with each duties with exceptional accuracy, whereas additionally indicating a considerable efficiency hole between AI-based fashions and Human professional in these domains.’
When it comes to effectivity, the AutoCompliance strategy took simply 53.1 seconds to run, in distinction to 2,418 seconds for equal human analysis on the identical duties.
Additional, the analysis run value $0.29 USD, in comparison with $207 USD for the human specialists. It must be famous, nevertheless, that that is primarily based on renting a GCP a2-megagpu-16gpu node month-to-month at a fee of $14,225 monthly – signifying that this sort of cost-efficiency is said primarily to a large-scale operation.
Dataset Investigation
For the evaluation, the researchers chosen 3,612 datasets combining the three,000 most-downloaded datasets from Hugging Face with 612 datasets from the 2023 Data Provenance Initiative.
The paper states:
‘Ranging from the three,612 goal entities, we recognized a complete of 17,429 distinctive entities, the place 13,817 entities appeared because the goal entities’ direct or oblique dependencies.
‘For our empirical evaluation, we take into account an entity and its license dependency graph to have a single-layered construction if the entity doesn’t have any dependencies and a multi-layered construction if it has a number of dependencies.
‘Out of the three,612 goal datasets, 2,086 (57.8%) had multi-layered buildings, whereas the opposite 1,526 (42.2%) had single-layered buildings with no dependencies.’
Copyrighted datasets can solely be redistributed with authorized authority, which can come from a license, copyright legislation exceptions, or contract phrases. Unauthorized redistribution can result in authorized penalties, together with copyright infringement or contract violations. Due to this fact clear identification of non-compliance is important.
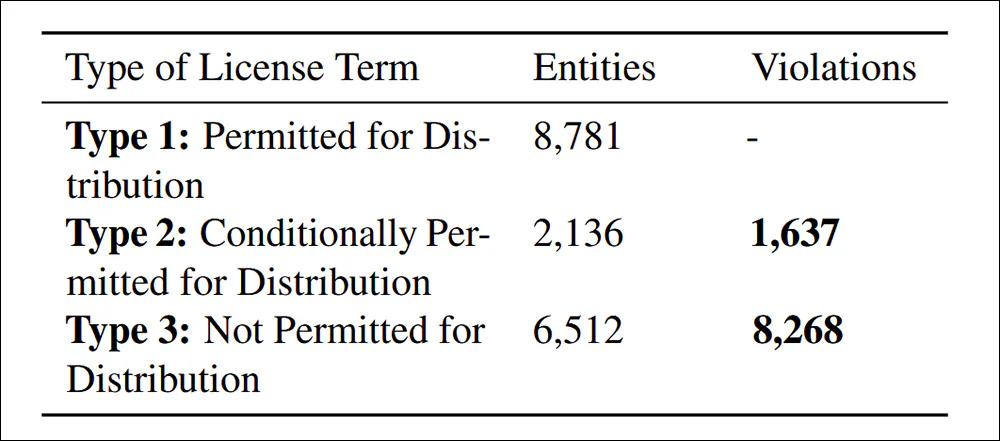
Distribution violations discovered underneath the paper’s cited Criterion 4.4. of Knowledge Compliance.
The research discovered 9,905 instances of non-compliant dataset redistribution, break up into two classes: 83.5% have been explicitly prohibited underneath licensing phrases, making redistribution a transparent authorized violation; and 16.5% concerned datasets with conflicting license situations, the place redistribution was allowed in concept however which didn’t meet required phrases, creating downstream authorized threat.
The authors concede that the chance standards proposed in NEXUS should not common and will fluctuate by jurisdiction and AI utility, and that future enhancements ought to give attention to adapting to altering world laws whereas refining AI-driven authorized evaluation.
Conclusion
It is a prolix and largely unfriendly paper, however addresses maybe the largest retarding consider present business adoption of AI – the chance that apparently ‘open’ information will later be claimed by numerous entities, people and organizations.
Below DMCA, violations can legally entail huge fines on a per-case foundation. The place violations can run into the thousands and thousands, as within the instances found by the researchers, the potential authorized legal responsibility is actually important.
Moreover, firms that may be confirmed to have benefited from upstream information can not (as usual) declare ignorance as an excuse, at the least within the influential US market. Neither do they presently have any life like instruments with which to penetrate the labyrinthine implications buried in supposedly open-source dataset license agreements.
The issue in formulating a system corresponding to NEXUS is that it will be difficult sufficient to calibrate it on a per-state foundation contained in the US, or a per-nation foundation contained in the EU; the prospect of making a really world framework (a form of ‘Interpol for dataset provenance’) is undermined not solely by the conflicting motives of the varied governments concerned, however the truth that each these governments and the state of their present legal guidelines on this regard are always altering.
* My substitution of hyperlinks for the authors’ citations.
† Six varieties are prescribed within the paper, however the last two should not outlined.
First printed Friday, March 7, 2025