Reinforcement Studying with Verifiable Rewards (RLVR) has confirmed efficient in enhancing LLMs’ reasoning and coding talents, notably in domains the place structured reference solutions permit clear-cut verification. This strategy depends on reference-based alerts to find out if a mannequin’s response aligns with a recognized right reply, sometimes by means of binary correctness labels or graded scores. RLVR has primarily been utilized to areas like math and coding, the place rule-based or tool-assisted verification is easy. Nevertheless, increasing RLVR to extra complicated and fewer structured duties has been troublesome as a result of challenges in verifying open-ended or ambiguous reference responses. Though generative fashions and closed-source LLMs like GPT-4o have been explored as verifiers, these options usually stay domain-specific and require intensive annotated datasets for coaching.
Current developments purpose to broaden RLVR purposes by introducing generative reward modeling, the place LLMs use their generative talents to provide judgments and justifications. These fashions may be educated with out detailed rationales, as a substitute counting on the arrogance of the verifier’s outputs to generate steady reward alerts. This method helps reinforcement studying in duties with noisy or ambiguous labels. Moreover, researchers are exploring RLVR in a greater diversity of domains utilizing extra free-form reference solutions—sourced from knowledgeable annotations and pretraining knowledge or generated by LLMs—transferring past narrowly outlined duties like math and logic puzzles. These efforts mark a big step towards scalable and domain-general RLVR coaching.
Tencent AI Lab and Soochow College researchers are exploring extending RLVR to complicated, unstructured domains like medication, chemistry, and schooling. They present that binary correctness judgments stay constant throughout LLMs when expert-written references can be found. To handle the restrictions of binary rewards in free-form duties, they introduce comfortable, generative model-based reward alerts. Utilizing compact 7B fashions, they prepare cross-domain reward verifiers with out requiring intensive domain-specific annotation. Their RLVR framework considerably outperforms prime open-source fashions in reasoning duties and scales successfully. In addition they launch a 570k-example dataset to help additional analysis in multi-domain RLVR.
The tactic makes use of expert-written reference solutions to information reward estimation for reinforcement studying. Responses are evaluated utilizing a generative LLM verifier, which outputs binary (0/1) or comfortable rewards based mostly on the chance of correctness. Rewards are normalized utilizing z-score normalization for steady coaching and higher studying dynamics. The authors prepare a compact (7B) generative reward mannequin utilizing judgments collected throughout RL exploration to keep away from relying solely on giant fashions. These binary labels are obtained from a bigger LLM and used to fine-tune the smaller verifier. This strategy balances efficiency and effectivity whereas rising robustness to noise and formatting variations.
The research makes use of two large-scale Chinese language QA datasets—one with 773k free-form math questions throughout college ranges and one other with 638k multi-subject college-level questions from ExamQA. These datasets function complicated, unstructured solutions that problem rule-based reward strategies. The researchers educated a 7B reward mannequin (RM-7B) utilizing 160k distilled samples and examined numerous RL approaches. Outcomes present that RL with model-based rewards outperforms rule-based strategies and supervised fine-tuning (SFT), particularly in reasoning duties. Notably, RM-7B achieves efficiency near the bigger 72B mannequin, highlighting its effectivity. Binary rewards outperform comfortable rewards in rule-based settings as a result of semantic mismatch points.
In conclusion, the research simplifies reward modeling by coaching a generative mannequin to output binary scores (1 or 0) with out counting on chain-of-thought reasoning. Whereas CoT aids in reasoning, its necessity for verifying semantic similarity stays unclear. In contrast to previous work that relied on format-based scoring, this strategy avoids strict reply formatting, decreasing handbook effort. The analysis extends RLVR past structured domains to areas like medication and economics, the place reference solutions are much less outlined. Utilizing a 7B mannequin, it exhibits that comfortable, model-based rewards improve efficiency in free-form duties, outperforming bigger fashions and enhancing RLVR’s adaptability and scalability.
Check out the Paper. All credit score for this analysis goes to the researchers of this undertaking. Additionally, be happy to comply with us on Twitter and don’t neglect to hitch our 85k+ ML SubReddit.
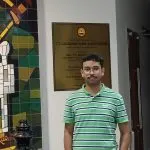
Sana Hassan, a consulting intern at Marktechpost and dual-degree scholar at IIT Madras, is captivated with making use of expertise and AI to deal with real-world challenges. With a eager curiosity in fixing sensible issues, he brings a contemporary perspective to the intersection of AI and real-life options.