Tactile sensing is a vital modality for clever methods to understand and work together with the bodily world. The GelSight sensor and its variants have emerged as influential tactile applied sciences, offering detailed details about contact surfaces by reworking tactile knowledge into visible photos. Nevertheless, vision-based tactile sensing lacks transferability between sensors on account of design and manufacturing variations, which end in vital variations in tactile indicators. Minor variations in optical design or manufacturing processes can create substantial discrepancies in sensor output, inflicting machine studying fashions skilled on one sensor to carry out poorly when utilized to others.
Laptop imaginative and prescient fashions have been broadly utilized to vision-based tactile photos on account of their inherently visible nature. Researchers have tailored illustration studying strategies from the imaginative and prescient neighborhood, with contrastive studying being widespread for creating tactile and visual-tactile representations for particular duties. Auto-encoding illustration approaches are additionally explored, with some researchers using Masked Auto-Encoder (MAE) to study tactile representations. Strategies like general-purpose multimodal representations make the most of a number of tactile datasets in LLM frameworks, encoding sensor sorts as tokens. Regardless of these efforts, present strategies typically require massive datasets, deal with sensor sorts as mounted classes, and lack the flexibleness to generalize to unseen sensors.
Researchers from the College of Illinois Urbana-Champaign proposed Sensor-Invariant Tactile Representations (SITR), a tactile illustration to switch throughout varied vision-based tactile sensors in a zero-shot method. It’s based mostly on the premise that reaching sensor transferability requires studying efficient sensor-invariant representations by publicity to numerous sensor variations. It makes use of three core improvements: using easy-to-acquire calibration photos to characterize particular person sensors with a transformer encoder, using supervised contrastive studying to emphasise geometric features of tactile knowledge throughout a number of sensors, and creating a large-scale artificial dataset that incorporates 1M examples throughout 100 sensor configurations.
Researchers used the tactile picture and a set of calibration photos for the sensor as inputs for the community. The sensor background is subtracted from all enter photos to isolate the pixel-wise colour adjustments. Following Imaginative and prescient Transformer (ViT), these photos are linearly projected into tokens, with calibration photos requiring tokenization solely as soon as per sensor. Additional, two supervision indicators information the coaching course of: a pixel-wise regular map reconstruction loss for the output patch tokens and a contrastive loss for the category token. Throughout pre-training, a light-weight decoder reconstructs the contact floor as a standard map from the encoder’s output. Furthermore, SITR employs Supervised Contrastive Studying (SCL), extending conventional contrastive approaches by using label info to outline similarity.
In object classification exams utilizing the researchers’ real-world dataset, SITR outperforms all baseline fashions when transferred throughout completely different sensors. Whereas most fashions carry out effectively in no-transfer settings, they fail to generalize when examined on distinct sensors. It exhibits SITR’s capacity to seize significant, sensor-invariant options that stay strong regardless of adjustments within the sensor area. In pose estimation duties, the place the purpose is to estimate 3-DoF place adjustments utilizing preliminary and ultimate tactile photos, SITR reduces the Root Imply Sq. Error by roughly 50% in comparison with baselines. Not like classification outcomes, ImageNet pre-training solely marginally improves pose estimation efficiency, exhibiting that options discovered from pure photos might not switch successfully to tactile domains for exact regression duties.
On this paper, researchers launched SITR, a tactile illustration framework that transfers throughout varied vision-based tactile sensors in a zero-shot method. They constructed large-scale, sensor-aligned datasets utilizing artificial and real-world knowledge and developed a way to coach SITR to seize dense, sensor-invariant options. The SITR represents a step towards a unified method to tactile sensing, the place fashions can generalize seamlessly throughout completely different sensor sorts with out retraining or fine-tuning. This breakthrough has the potential to speed up developments in robotic manipulation and tactile analysis by eradicating a key barrier to the adoption and implementation of those promising sensor applied sciences.
Try the Paper and Code. All credit score for this analysis goes to the researchers of this mission. Additionally, be at liberty to comply with us on Twitter and don’t overlook to hitch our 85k+ ML SubReddit.
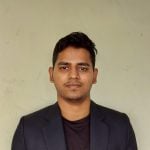
Sajjad Ansari is a ultimate 12 months undergraduate from IIT Kharagpur. As a Tech fanatic, he delves into the sensible functions of AI with a deal with understanding the affect of AI applied sciences and their real-world implications. He goals to articulate advanced AI ideas in a transparent and accessible method.