Giant Language fashions (LLMs) function by predicting the following token primarily based on enter information, but their efficiency suggests they course of info past mere token-level predictions. This raises questions on whether or not LLMs interact in implicit planning earlier than producing full responses. Understanding this phenomenon can result in extra clear AI programs, enhancing effectivity and making output era extra predictable.
One problem in working with LLMs is predicting how they are going to construction responses. These fashions generate textual content sequentially, making controlling the general response size, reasoning depth, and factual accuracy difficult. The dearth of specific planning mechanisms implies that though LLMs generate human-like responses, their inner decision-making stays opaque. In consequence, customers typically depend on immediate engineering to information outputs, however this methodology lacks precision and doesn’t present perception into the mannequin’s inherent response formulation.
Current strategies to refine LLM outputs embody reinforcement studying, fine-tuning, and structured prompting. Researchers have additionally experimented with resolution timber and exterior logic-based frameworks to impose construction. Nonetheless, these strategies don’t absolutely seize how LLMs internally course of info.
The Shanghai Synthetic Intelligence Laboratory analysis staff has launched a novel method by analyzing hidden representations to uncover latent response-planning behaviors. Their findings counsel that LLMs encode key attributes of their responses even earlier than the primary token is generated. The analysis staff examined their hidden representations and investigated whether or not LLMs interact in emergent response planning. They launched easy probing fashions educated on immediate embeddings to foretell upcoming response attributes. The research categorized response planning into three essential areas: structural attributes, akin to response size and reasoning steps, content material attributes together with character selections in story-writing duties, and behavioral attributes, akin to confidence in multiple-choice solutions. By analyzing patterns in hidden layers, the researchers discovered that these planning skills scale with mannequin dimension and evolve all through the era course of.
To quantify response planning, the researchers performed a sequence of probing experiments. They educated fashions to foretell response attributes utilizing hidden state representations extracted earlier than output era. The experiments confirmed that probes might precisely predict upcoming textual content traits. The findings indicated that LLMs encode response attributes of their immediate representations, with planning skills peaking in the beginning and finish of responses. The research additional demonstrated that fashions of various sizes share comparable planning behaviors, with bigger fashions exhibiting extra pronounced predictive capabilities.
The experiments revealed substantial variations in planning capabilities between base and fine-tuned fashions. Nice-tuned fashions exhibited higher prediction accuracy in structural and behavioral attributes, confirming that planning behaviors are strengthened via optimization. As an example, response size prediction confirmed excessive correlation coefficients throughout fashions, with Spearman’s correlation reaching 0.84 in some instances. Equally, reasoning step predictions exhibited robust alignment with ground-truth values. Classification duties akin to character alternative in story writing and multiple-choice reply choice carried out considerably above random baselines, additional supporting the notion that LLMs internally encode parts of response planning.
Bigger fashions demonstrated superior planning skills throughout all attributes. Throughout the LLaMA and Qwen mannequin households, planning accuracy improved persistently with elevated parameter rely. The research discovered that LLaMA-3-70B and Qwen2.5-72B-Instruct exhibited the best prediction efficiency, whereas smaller fashions like Qwen2.5-1.5B struggled to encode long-term response constructions successfully. Additional, layer-wise probing experiments indicated that structural attributes emerged prominently in mid-layers, whereas content material attributes grew to become extra pronounced in later layers. Behavioral attributes, akin to reply confidence and factual consistency, remained comparatively steady throughout completely different mannequin depths.
These findings spotlight a elementary side of LLM habits: they don’t merely predict the following token however plan broader attributes of their responses earlier than producing textual content. This emergent response planning skill has implications for enhancing mannequin transparency and management. Understanding these inner processes may help refine AI fashions, main to higher predictability and decreased reliance on post-generation corrections. Future analysis might discover integrating specific planning modules inside LLM architectures to boost response coherence and user-directed customization.
Check out the Paper. All credit score for this analysis goes to the researchers of this venture. Additionally, be at liberty to observe us on Twitter and don’t overlook to hitch our 75k+ ML SubReddit.
🚨 Really helpful Learn- LG AI Analysis Releases NEXUS: An Superior System Integrating Agent AI System and Knowledge Compliance Requirements to Deal with Authorized Considerations in AI Datasets
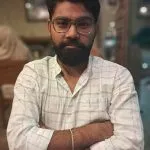
Nikhil is an intern guide at Marktechpost. He’s pursuing an built-in twin diploma in Supplies on the Indian Institute of Know-how, Kharagpur. Nikhil is an AI/ML fanatic who’s at all times researching purposes in fields like biomaterials and biomedical science. With a powerful background in Materials Science, he’s exploring new developments and creating alternatives to contribute.