Multi-agent techniques (MAS) are pivotal in synthetic intelligence, enabling a number of brokers to work collaboratively to resolve intricate duties. These techniques are designed to operate in dynamic and unpredictable environments, addressing information evaluation, course of automation, and decision-making duties. By incorporating superior frameworks and leveraging giant language fashions (LLMs), MAS has elevated effectivity and flexibility for numerous functions. Nonetheless, enhancing their capability to deal with real-world complexities stays a big problem.
A persistent situation in conventional MAS is their restricted flexibility and flexibility. These techniques usually battle with dynamic activity necessities, counting on inflexible activity allocation and predefined unsuitable procedures for altering situations. This rigidity will increase the chance of errors and limits the system’s capability to get better successfully when deviations happen. Furthermore, the shortage of built-in mechanisms for self-planning and error correction exacerbates these inefficiencies, resulting in wasted sources and suboptimal efficiency in complicated situations.
Current strategies for MAS growth embrace frameworks comparable to LangChain and AgentScope, which offer activity allocation and growth instruments. Whereas these frameworks facilitate the creation of brokers and streamline deployment, they’re restricted by their incapability to handle numerous information situations or present sturdy options for superior analytics. For instance, conventional MAS techniques like MetaGPT and AutoAgents lack world monitoring mechanisms and versatile agent era, rendering them ineffective for duties requiring dynamic changes and complete error correction throughout execution.
Researchers from Ant Group and JD Group have launched ROMAS, a Position-Primarily based Multi-Agent System designed to handle these limitations. ROMAS is constructed on the DB-GPT framework and incorporates role-based collaboration, enabling brokers to tackle particular roles comparable to planners, displays, and employees. This revolutionary system facilitates real-time activity monitoring, adaptive error correction, and low-code growth. ROMAS enhances effectivity and scalability in database monitoring and planning duties by supporting seamless deployment throughout numerous situations.
The ROMAS methodology emphasizes adaptability and robustness by means of its three operational phases: initialization, execution, and re-planning. Within the initialization section, the system divides duties into subtasks and assigns them to specialised brokers, every with distinct roles like information extraction, retrieval, and evaluation. Throughout execution, brokers collaborate to finish duties primarily based on predefined methods. A self-monitoring mechanism permits brokers to determine and handle errors dynamically, with unresolved points escalated to a monitor for additional evaluation. The re-planning section refines methods utilizing insights from the earlier phases, making certain alignment with the system’s aims. The DB-GPT framework underpins ROMAS with highly effective database dealing with, reminiscence categorization, and self-reflection capabilities, permitting for efficient activity completion even in complicated environments.
The researchers performed in depth evaluations to display ROMAS’s efficiency, utilizing datasets like FAMMA and HotpotQA to check its capabilities in domain-specific and normal situations. On the FAMMA dataset, ROMAS achieved a hit charge of 81.68%, whereas on the HotpotQA dataset, it reached 85.24%. These outcomes spotlight its superior efficiency to different MAS techniques, together with Generative Brokers and AutoAgents. Marked options just like the monitor mechanism and reminiscence categorization contributed considerably to this success. The research additionally revealed that ROMAS decreased growth complexity, with code quantity reducing to 1,500 rows in comparison with 2,500 rows in LangChain and 1,800 in AgentScope. Additional, ROMAS demonstrated a mean question processing time of 12.23 seconds, considerably sooner than its counterparts.
Key findings embrace ROMAS’s capability to handle pipeline and logical errors successfully. For example, the system’s error correction mechanisms decreased error impression charges by 22.66% on common, showcasing its sturdy problem-solving capabilities. Integrating superior reminiscence mechanisms and the DB-GPT framework enhanced activity effectivity by enabling seamless transitions between operational phases. These options enhance system reliability and be sure that ROMAS maintains excessive adaptability throughout numerous situations.
In conclusion, ROMAS represents a big development in multi-agent techniques by addressing the essential limitations of conventional frameworks. Developed by Ant Group and JD Group researchers, the system leverages role-based collaboration, self-monitoring, and low-code deployment to streamline database monitoring and planning duties. ROMAS has demonstrated superior efficiency by means of in depth evaluations, providing a scalable and environment friendly answer for complicated analytical challenges. This innovation paves the way in which for additional developments in clever multi-agent techniques and their functions.
Take a look at the Paper. All credit score for this analysis goes to the researchers of this venture. Additionally, don’t overlook to comply with us on Twitter and be part of our Telegram Channel and LinkedIn Group. Don’t Overlook to affix our 60k+ ML SubReddit.
🚨 Trending: LG AI Analysis Releases EXAONE 3.5: Three Open-Supply Bilingual Frontier AI-level Fashions Delivering Unmatched Instruction Following and Lengthy Context Understanding for World Management in Generative AI Excellence….
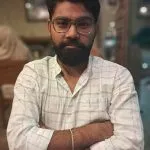
Nikhil is an intern guide at Marktechpost. He’s pursuing an built-in twin diploma in Supplies on the Indian Institute of Expertise, Kharagpur. Nikhil is an AI/ML fanatic who’s at all times researching functions in fields like biomaterials and biomedical science. With a robust background in Materials Science, he’s exploring new developments and creating alternatives to contribute.