Transformers have emerged as foundational instruments in machine studying, underpinning fashions that function on sequential and structured knowledge. One essential problem on this setup is enabling the mannequin to know the place of tokens or inputs since Transformers inherently lack a mechanism for encoding order. Rotary Place Embedding (RoPE) turned a preferred resolution, particularly in language and imaginative and prescient duties, as a result of it effectively encodes absolute positions to facilitate relative spatial understanding. As these fashions develop in complexity and utility throughout modalities, enhancing the expressiveness and dimensional flexibility of RoPE has turn out to be more and more important.
A major problem arises when scaling RoPE, from dealing with easy 1D sequences to processing multidimensional spatial knowledge. The issue lies in preserving two important options: relativity—enabling the mannequin to differentiate positions relative to 1 one other—and reversibility—making certain distinctive restoration of unique positions. Present designs typically deal with every spatial axis independently, failing to seize the interdependence of dimensions. This method results in an incomplete positional understanding in multidimensional settings, proscribing the mannequin’s efficiency in complicated spatial or multimodal environments.
Efforts to increase RoPE have typically concerned duplicating 1D operations alongside a number of axes or incorporating learnable rotation frequencies. A standard instance is commonplace 2D RoPE, which independently applies 1D rotations throughout every axis utilizing block-diagonal matrix types. Whereas sustaining computational effectivity, these methods can not symbolize diagonal or mixed-directional relationships. Not too long ago, learnable RoPE formulations, equivalent to STRING, tried so as to add expressiveness by instantly coaching the rotation parameters. Nevertheless, these lack a transparent mathematical framework and don’t assure that the elemental constraints of relativity and reversibility are happy.
Researchers from the College of Manchester launched a brand new methodology that systematically extends RoPE into N dimensions utilizing Lie group and Lie algebra idea. Their method defines legitimate RoPE constructions as these mendacity inside a maximal abelian subalgebra (MASA) of the particular orthogonal Lie algebra so(n). This technique brings a beforehand absent theoretical rigor, making certain the positional encodings meet relativity and reversibility necessities. Quite than stacking 1D operations, their framework constructs a foundation for position-dependent transformations that may flexibly adapt to increased dimensions whereas sustaining mathematical ensures.
The core methodology defines the RoPE transformation as a matrix exponential of skew-symmetric turbines inside the Lie algebra so(n). For traditional 1D and 2D instances, these matrices produce conventional rotation matrices. The novelty is available in generalizing to N dimensions, the place the researchers choose a linearly impartial set of N turbines from a MASA of so(d). This ensures that the ensuing transformation matrix encodes all spatial dimensions reversibly and comparatively. The authors show that this formulation, particularly the usual ND RoPE, corresponds to the maximal toral subalgebra—a construction that divides the enter house into orthogonal two-dimensional rotations. To allow dimensional interactions, the researchers incorporate a learnable orthogonal matrix, Q, which modifies the premise with out disrupting the mathematical properties of the RoPE building. A number of methods for studying Q are proposed, together with the Cayley remodel, matrix exponential, and Givens rotations, every providing interpretability and computational effectivity trade-offs.
The tactic demonstrates strong theoretical efficiency, proving that the constructed RoPE retains injectivity inside every embedding cycle. When dimensionality d² equals the variety of dimensions N, the usual foundation effectively helps structured rotations with out overlap. For increased values of d, extra versatile turbines will be chosen to accommodate multimodal knowledge higher. The researchers confirmed that matrices like B₁ and B₂ inside so(6) might symbolize orthogonal and impartial rotations throughout six-dimensional house. Though no empirical outcomes have been reported for downstream activity efficiency, the mathematical construction confirms that each key properties—relativity, and reversibility—are preserved even when introducing realized inter-dimensional interactions.
This analysis from the College of Manchester provides a mathematically full and stylish resolution to the constraints of present RoPE approaches. The analysis closes a big hole in positional encoding by grounding their methodology in algebraic idea and providing a path to be taught inter-dimensional relationships with out sacrificing foundational properties. The framework applies to conventional 1D and 2D inputs and scales to extra complicated N-dimensional knowledge, making it a foundational step towards extra expressive Transformer architectures.
Take a look at Paper. All credit score for this analysis goes to the researchers of this challenge. Additionally, be at liberty to observe us on Twitter and don’t neglect to affix our 90k+ ML SubReddit.
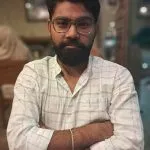
Nikhil is an intern guide at Marktechpost. He’s pursuing an built-in twin diploma in Supplies on the Indian Institute of Know-how, Kharagpur. Nikhil is an AI/ML fanatic who’s at all times researching functions in fields like biomaterials and biomedical science. With a powerful background in Materials Science, he’s exploring new developments and creating alternatives to contribute.